Can AI Help Us Decipher Lost Languages?
That depends mainly on the reasons we haven’t yet deciphered ancient textsAI may certainly help scholars with the ancient Mesopotamia languages. Many thousands of undeciphered documents written with styluses on clay tablets lie waiting very, very patiently for someone who has the time:
Developed more than 5,000 years ago in Mesopotamia, the land between the Tigris and Euphrates rivers where modern-day Iraq now lies, cuneiform captured life in a complex and fascinating civilisation for some three millennia. From furious letters between warring royal siblings to rituals for soothing a fractious baby, the tablets offer a unique insight into a society at the dawn of history.
They chronicle the rise of fall of Akkad, Assyria and Babylonia, the world’s first empires. An estimated half a million of them have been excavated, and more are still buried in the ground.
Sophie Hardach, “The key to cracking long-dead languages?” at BBC (December 9, 2018)
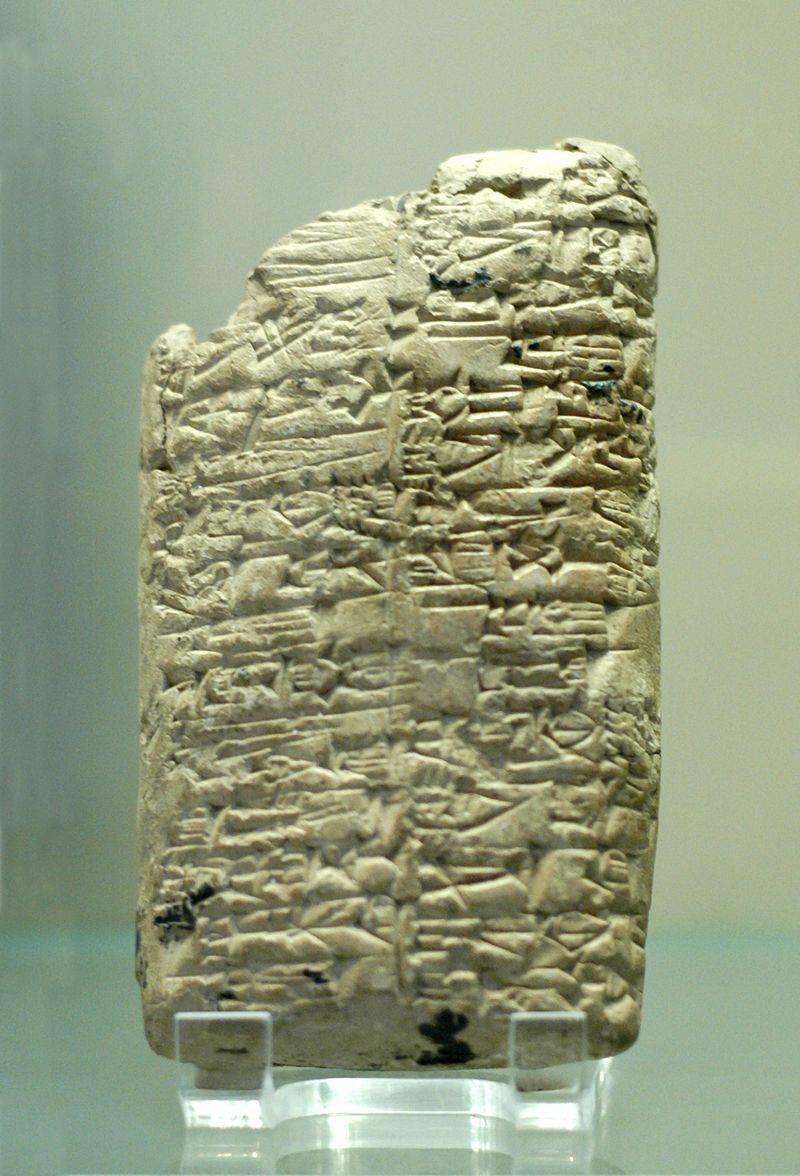
The tablet above, deciphered, commemorates the victories of Rimush, king of Akkad, about 2250 BCE.
Ninety percent of such documents are untranslated because it is a time-consuming job for the few scholars who know the language. One project proposes to speed up translation of 69,000 Mesopotamian administrative records from the 21 century BC:
“The texts we’re working on are not very interesting individually, but they’re extremely interesting if you take them as groups of texts,” says Pagé-Perron, who expects the English versions to be online within the next year. The records give us a picture of day to day life in ancient Mesopotamia, of power structures and trading networks, but also of other aspects of its social history, such as the role of female workers. Searchable translations would enable researchers from other areas to explore these rich facets of life in the ancient world.
Sophie Hardach, “The key to cracking long-dead languages?” at BBC (December 9, 2018)
The machine-learning approach works with a number of assumptions that might help decipher related but unknown languages as well:
A word like “overloading,” for instance, has both a prefix — “over” — and a suffix — “ing.” The system would anticipate that other words in the language will feature the prefix “over” or the suffix “ing” or both, and that a cognate of “overloading” in another language — say, “surchargeant” in French — would have a similar three-part structure.
Larry Hardesty, “Computer automatically deciphers ancient language” at MIT News (June 30, 2010)
But some challenges are much harder. For example, in 1953, cryptographer Michael Ventris (1922–1956) broke Minoan B. Minoan B (sometimes called Linear B) was a language used in Crete after 1400 BC, when the island was conquered by the Mycenaean civilization.
But Minoan A (Linear A), a language whose inscriptions date from 1800–1400 BC, remains undeciphered to this day. Behind it may well stretch a succession of languages going back thousands of years. Can AI help?
Well, first, what exactly did Ventris do? Studying the artifacts, originally found in 1886 by British archeologist Arthur Evans, he made two guesses:
First, Ventris conjectured that many of the repeated words in the Linear B vocabulary were names of places on the island of Crete. That turned out to be correct.
His second breakthrough was to assume that the writing recorded an early form of ancient Greek. That insight immediately allowed him to decipher the rest of the language. In the process, Ventris showed that ancient Greek first appeared in written form many centuries earlier than previously thought.
Emerging Technology from the arXiv, “Machine learning has been used to automatically translate long-lost languages” at Technology Review
Even if we don’t have any idea what an inscription in Minoan A, such as the one (below) from an inner surface of a cup, means, we can begin by inferring some things:
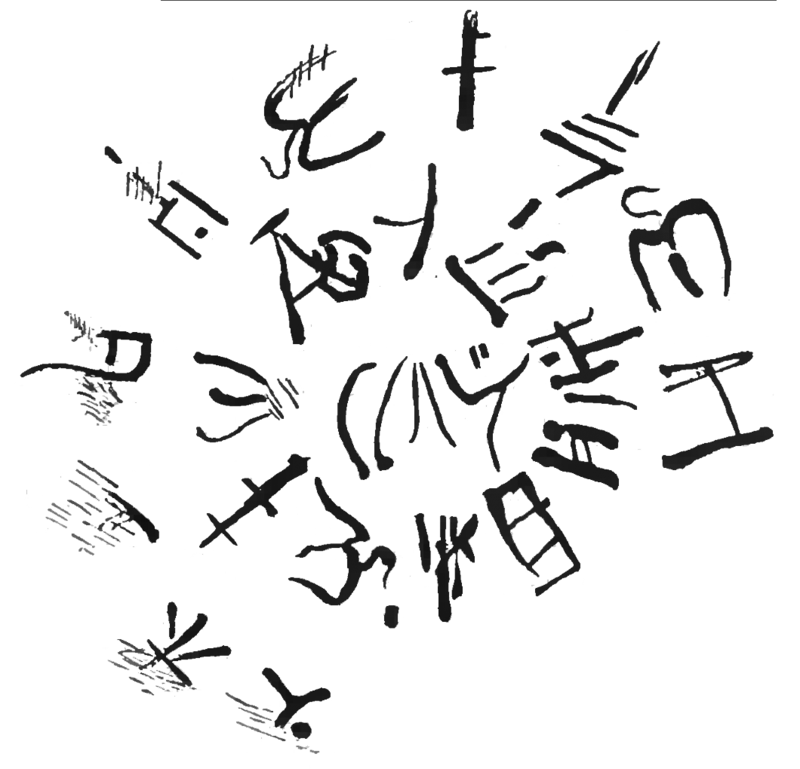
First, human languages are about things that matter to people. We might read about the “king,” “son,” “harvest,” or “born”. Once we have deciphered one instance clearly, many others open up, maybe a whole sentence: “The king’s son was born at harvest time.” That would illuminate sentences in other documents and point to grammatical constructions that can help us decipher other words (“The king had three sons and two [?]” Probably, “daughters”).
Second, to create meaning we follow logical patterns, which means ruling out most possibilities. For example, we might read: “Jan Ma walked over and sat on the sofa” in English or Chinese but we would not likely read in either language that “The sofa walked over and sat on Jan Ma.”
Using rules derived from such principles and others, machine translation can help speed things up even with smaller databases of text, as a research team showed:
Now Luo and co have gone further to show how machine translation can decipher languages that have been lost entirely. The constraint they use has to do with the way languages are known to evolve over time.
The idea is that any language can change in only certain ways—for example, the symbols in related languages appear with similar distributions, related words have the same order of characters, and so on. With these rules constraining the machine, it becomes much easier to decipher a language, provided the progenitor language is known.
Emerging Technology from the arXiv, “Machine learning has been used to automatically translate long-lost languages” at Technology Review
The researchers succeeded in redeciphering Minoan B (67.3% efficiency) and Ugaritic, an early version of Hebrew discovered in 1929.
So why not just apply this method to Minoan A?
Because no one knows what type of language Minoan A represents. Machine learning methods depend on having a language to compare the unknown language to.
Suppose, for example, you have a document in English and you are presented with an unrelated document in the related language, Dutch. The ways English and Dutch changed and moved away from each other over the years enable good guesses as to what words in Dutch might mean. Machine learning could make the process much faster.
It might even help if we knew what the Minoan A writings refer to. For example, accounts of the outcome of the 2019 Tour de France in Spanish and Hungarian might help us decipher an account of the same outcome in Mandarin because the story can only mean certain specific things. But for Minoan A, we do not have anything as specific as that, at least not yet.
Some are skeptical that any type of AI can help, absent such a breakthrough:
“If the authors believe that their approach will eventually lead to the computerised ‘automatic’ decipherment of currently undeciphered scripts,” he writes in an e-mail, “then I am afraid I am not at all persuaded by their paper.” The researchers’ approach, he says, presupposes that the language to be deciphered has an alphabet that can be mapped onto the alphabet of a known language — “which is almost certainly not the case with any of the important remaining undeciphered scripts,” Robinson writes. It also assumes, he argues, that it’s clear where one character or word ends and another begins, which is not true of many deciphered and undeciphered scripts.
Larry Hardesty, “Computer automatically deciphers ancient language” at MIT News (June 30, 2010)
But new archeological findings turn up frequently. If we do find the needed clues to Minoan A and other lost languages, AI will certainly get the results to archeology students much more quickly.
Note: It was the Mesopotamians who gave us the sixty-second minute and the sixty-minute hour, a departure from our usual decimal (5s and 10s) counting system, among many other firsts.
More on AI, archeology, and literature:
Does AI challenge Biblical archeology? Sadly, many surviving documents are so damaged that they cannot be read using traditional methods. AI can sometimes be used to decipher them.
and
Can AI prove that Shakespeare had ghostwriters? An author’s unique style is like a fingerprint. AI can fill it in Turning AI loose on some of these vexing problems should give literary scholars more to write about rather than less. The AI verdict may not always be right but it is bound to be food for thought.