Presidential Pundits—a P-Hacking Parable
In politics, as elsewhere, too many studies flop when other researchers attempt to replicate them with fresh dataHere we go again. Every four years, we have a presidential election and, every four years, “experts” re-emerge using their semi-plausible models to tell us who the winner will be. The models are, in reality, dodgy. The main takeaway is a compelling demonstration of the allure and pitfalls of what statisticians call p-hacking (torturing the data to get “statistically significant” results). The pressure to publish, not perish, seduces too many researchers into p-hacking, which fuels the replication crisis that is undermining the credibility of science and scientists—too many studies flop when others attempt to replicate them with fresh data.
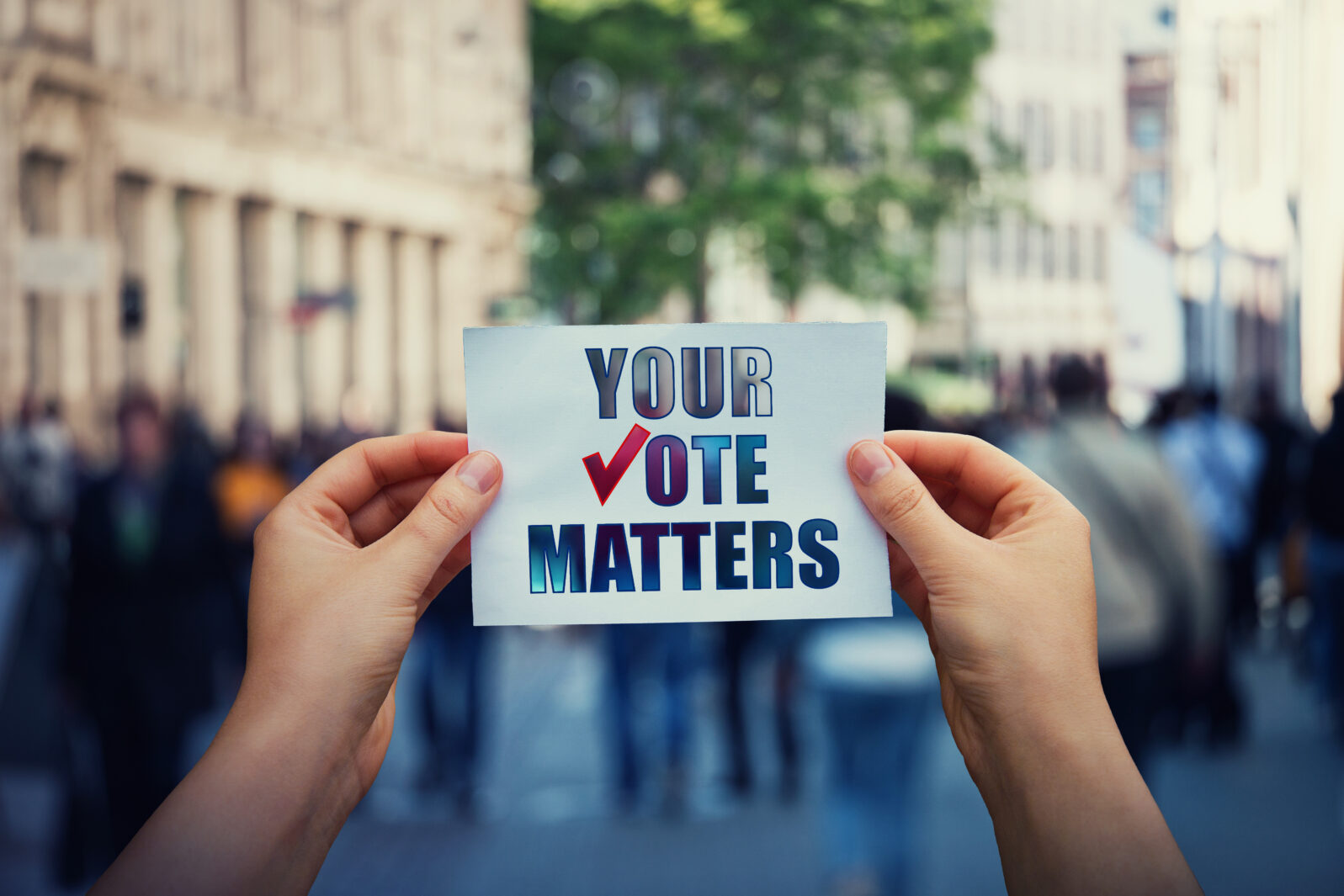
For example, many Asian cultures celebrate the Harvest Moon Festival on the night of the fifteenth day of the eighth moon of the lunar calendar. That is the brightest full moon of the year and it occurs during the harvest season in the northern hemisphere. Families customarily gather for bonding and celebration and to eat a festival meal that includes traditional moon cakes outdoors in the moonlight at midnight.
A researcher who had written several questionable papers supporting the idea that people can postpone death until after important ceremonies reported that elderly Chinese women can postpone their deaths until after the Harvest Moon Festival. The paper was published in the Journal of the American Medical Association in 1990. Its results were based on data for the years 1960–1984 showing that Chinese-American women who were 75 and older experienced fewer deaths in the week preceding the festival than the one after—if deaths on the festival day are counted as having occurred after the festival.
Consider all the possible p-hacks
Why 1960–1984 in a paper published in 1990? Why Chinese-Americans? Why women? Why 75 and older? Why count deaths that occurred on the festival day, before mooncakes at midnight, as successful death postponements? Attempts to replicate the results using 1985–2000 data for Chinese-, Korean-, and Vietnamese-American women who were 75 and older found that no group experienced more deaths after the festival than before.
Which brings us to presidential-prediction p-hacking. I will discuss one example here and another in a future post.
Helmut Norpoth, a political science professor at Stony Brook University, is reliably in the news before each presidential election, sharing his model’s predictions. His model uses just three variables: a presidential voting cycle, long-term trends in partisanship, and the two candidates’ performance in the primaries.
The Primary Model
He calls his model the Primary Model because the most important factor is how the candidates fared in the primary elections before becoming their parties’ candidates — in particular, the outcomes of the “early primaries” in New Hampshire and South Carolina. These are, in fact, not the earliest primaries nor has he always used these two primaries.
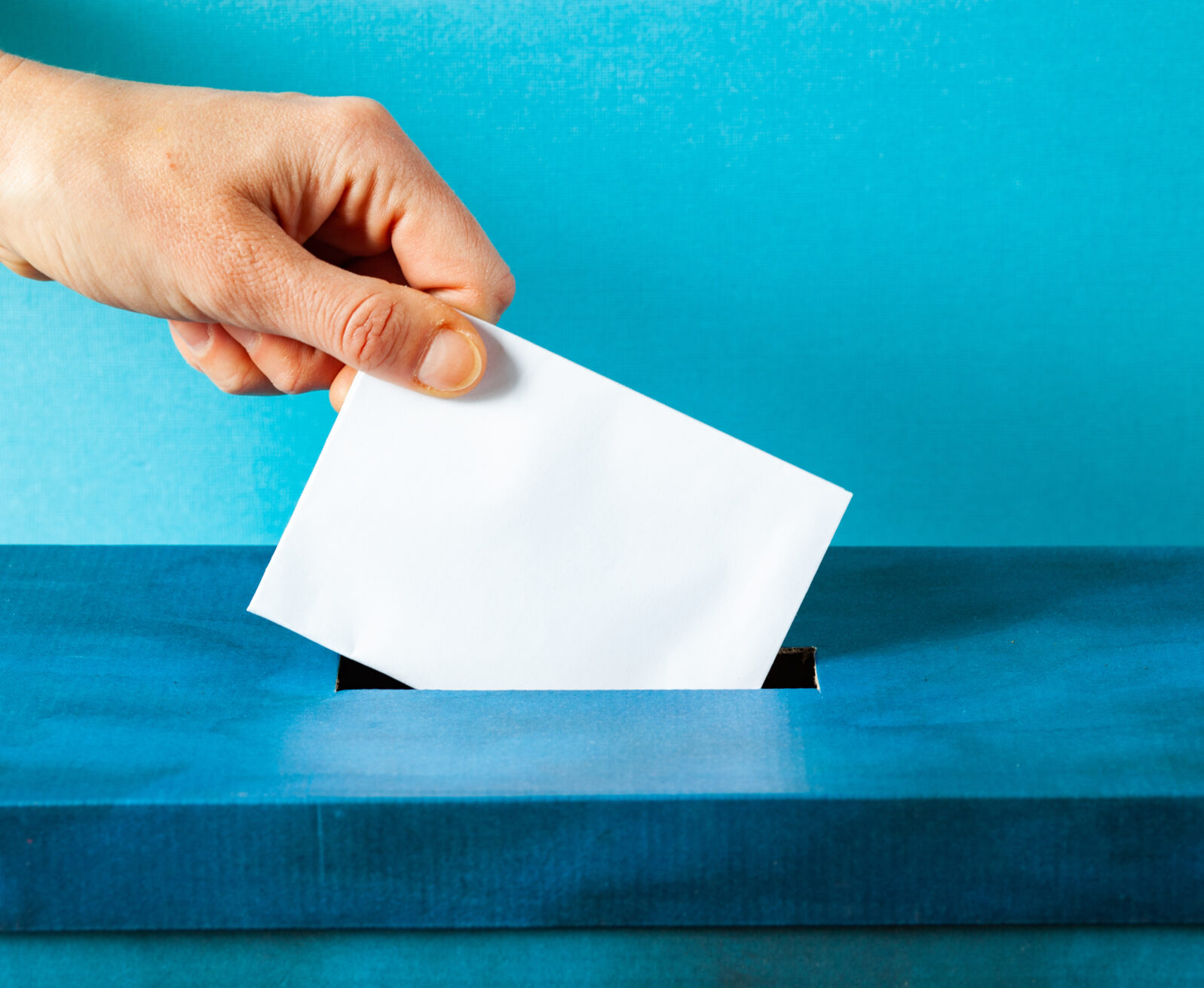
In the 2024 election cycle, New Hampshire (January 23) and South Carolina (February 24) were the earliest Republican primaries, though Michigan was just three days after South Carolina. On the Democratic side, the first primaries were South Carolina (February 3), Nevada (February 6), and Michigan (February 27). The Democratic New Hampshire primary was not until April 27.
Norpoth’s original Primary Model, introduced in 1996, used all presidential primaries for the elections from 1912 through 1948 and only the New Hampshire primary for 1952 through 1992. It correctly predicted the winners of 20 of the 21 primaries from 1912 through 1992. These were, of course, not really predictions since the elections had already happened. Why did he switch in 1952 from all primaries to New Hampshire only? The all-primary model obviously stopped making correct predictions in 1948 and the New Hampshire switch “solved” that problem.
But wait, there’s more
In 2012, the Primary Model switched again, from only New Hampshire to both New Hampshire and South Carolina, evidently because adding South Carolina helped the model make predictions of the 2008 election that had already occurred.
That year, John McCain, the eventual Republican nominee for president, won the Republican New Hampshire primary with 37.2% of the vote. Meanwhile, Barack Obama, the eventual Democratic nominee, finished second in the Democratic primary with 36.4% of the vote, behind Hillary Clinton’s 39.2%—suggesting that McCain would beat Obama. With various adjustments, Norpoth got his model to predict that Obama would get a narrow 50.1% of the votes. (Obama won a comfortable 52.9%.) In retrospect, the Primary Model would have worked better if it had included the South Carolina primaries, where Obama won his with 55.4% while McCain won his with 33.2%. So, Norpoth added South Carolina to the model. How did that work out? Mixed, as might be expected.
In 2016 he wrote that, “it never ceases to amaze [me] how many students of elections are surprised to learn that presidential primaries predict anything beyond perhaps who wins the nomination. Yet the outcomes of these primaries prove to be uncanny leading indicators of wins the general election for president in November.” His uncanny 2016 prediction (with 87% to 99% certainty): “Donald Trump will defeat Hillary Clinton with 52.5% of the two-party popular vote, with her getting 47.5%.” Trump lost the popular vote by 2.3 percentage points but got the most electoral college votes and Norpoth counted that as a correct prediction, even though the model was explicitly advertised as predicting the former, not the latter.
Modifying the Primary Model
After that incorrect (but counted as correct) prediction in 2016, the Primary Model was modified to predict the electoral vote rather than the popular vote. It didn’t help. In 2020 Norpoth’s uncanny prediction was that Trump had a 91% to 95% probability of winning re-election and would most likely win 362 electoral votes to Joe Biden’s 176 votes.
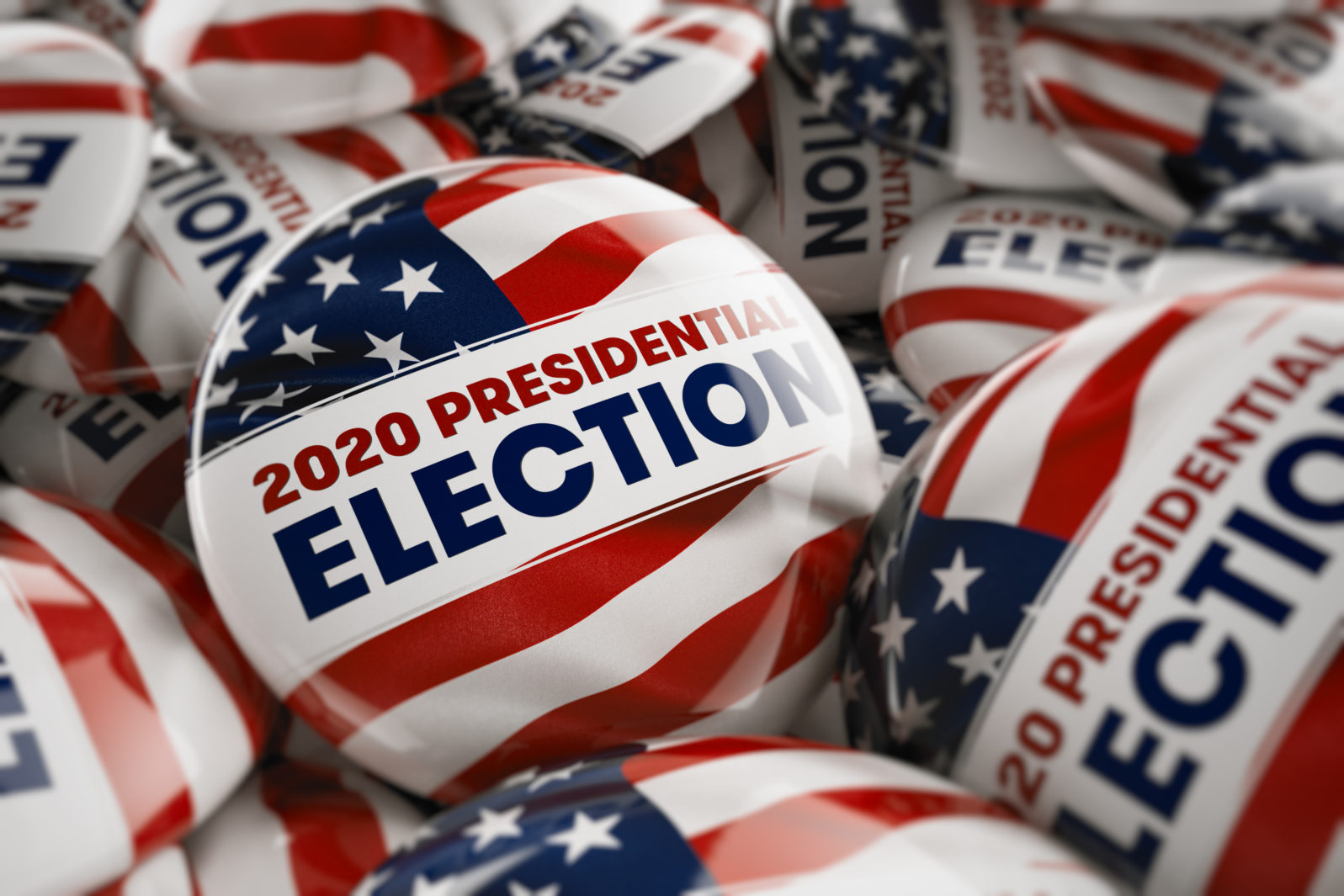
The Stony Brook University newspaper reported that his Trump-victory prediction was unconditional and unaffected by current events:
While some might suspect that unusual circumstances — e.g., the COVID-19 pandemic and the civil unrest in the wake of the George Floyd killing — might have an unpredictable effect on the election results, Norpoth said those crises have no bearing on his projection.
“My prediction is what I call ‘unconditional final,’” he said. “It does not change. It’s a mathematical model based on things that have happened.”
After Biden defeated Trump by 306 to 232 electoral votes, Norpoth attributed his wild miss to “a perfect storm” of current events that he had previously dismissed as irrelevant:
the outbreak of the coronavirus pandemic; then, triggered by the lockdown aimed to keep people safe, came an economic downturn of a scale not seen since the Great Depression; then, with the same goal in mind, came an unprecedented expansion of voting by mail; add to that the killing of George Floyd, which sparked a wave of racial unrest not seen since the 1960’s.
Why the wild miss?
Norpoth is no doubt well-intentioned but his Primary Model was created during a time when many well-intentioned researchers did not realize the perils of p-hacking. They thought that this is how research is done. Do whatever you can to obtain statistical significance and report the results. We now know better.
Oh, what about the upcoming election? There is the not-so-slight problem that Kamala Harris wasn’t on the ballot in the New Hampshire or South Carolina primaries — and Joe Biden was a write-in candidate in New Hampshire. But forecasters want to forecast, so Norpoth counted Biden’s votes as Harris votes and concluded that Harris has a 75% probability of defeating Donald Trump, with 315 electoral votes for her and 223 for Trump. If not, the model can always be p-hacked again.