Programmer: Deep Learning Can’t Give Us Computers That Think
Artificial general intelligence (AGI) — computers that think like humans — would need to be able to generalize, which he says Deep Learning doesn’t doAt Medium late last year, a programmer/researcher who writes under the name “Rebel Science,” assessed Deep Learning, a favorite of the self-driving car quest, as “useless” because it can’t generalize:
The biggest problem with DL is its inherent inability to effectively generalize. Without generalization, edge cases are an insurmountable problem, something that the autonomous vehicle industry found out the hard way after wasting more than $100 billion by betting on DL.
Rebel Science, “Deep Learning Is Not Just Inadequate for Solving AGI, It Is Useless” at Medium (Nov 2, 2022)
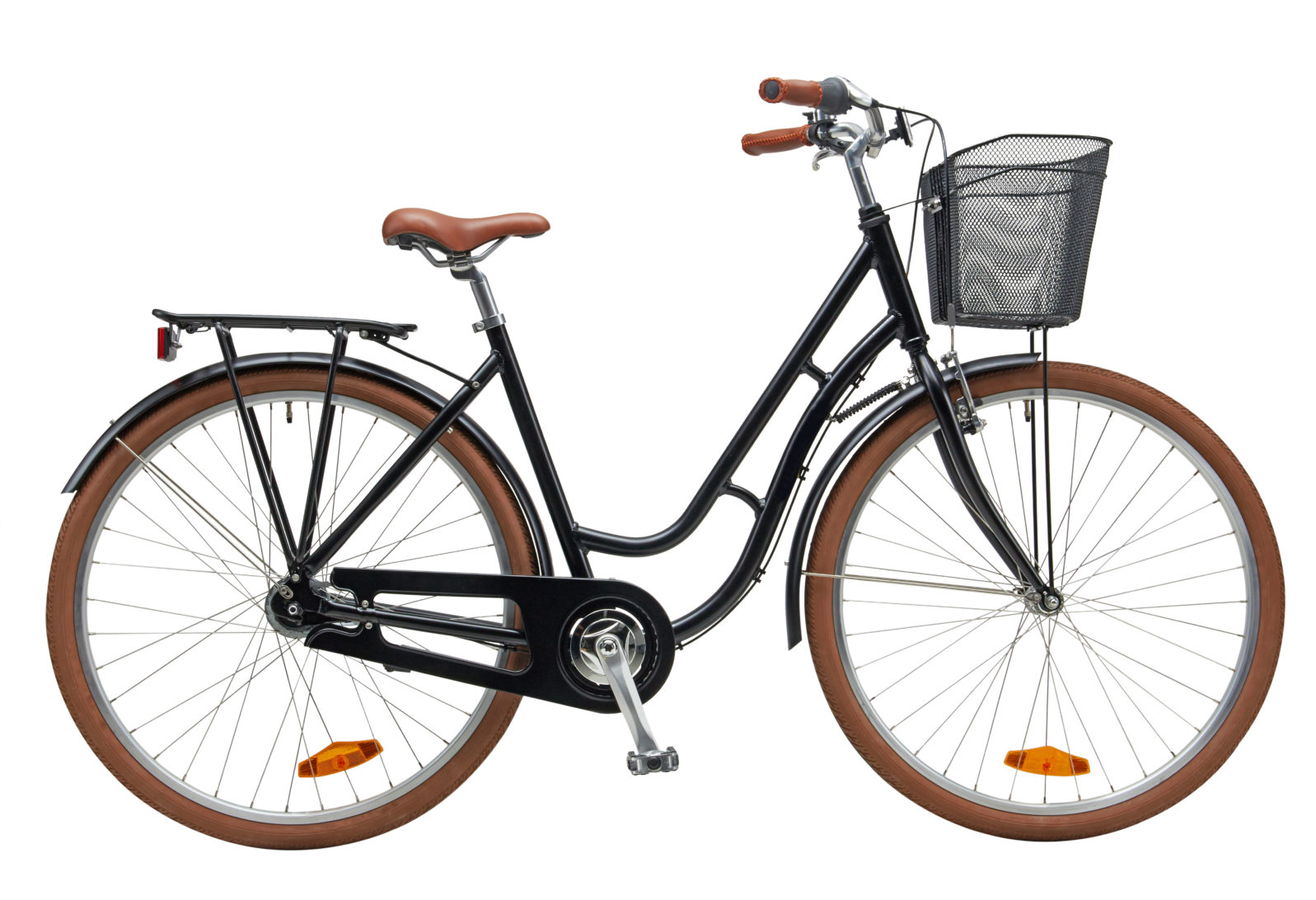
Displaying for readers a picture of a bicycle, he continues,
A deep neural network cannot perceive this bicycle unless it has been previously trained to recognize it.
Generalization is the ability of an intelligent system to perceive any object or pattern without recognizing it. An Amazon Indian, for example, can instantly perceive a bicycle even if he has never seen one before. He can instantly see its 3D shape, size, borders, colors, its various parts, its position relative to other objects, whether it is symmetrical, opaque, transparent or partially occluding, etc. He can perceive all these things because his brain has the ability to generalize. Moreover, his perception of the bicycle is automatically invariant to transformations in his visual field…
With DL, perception is impossible without recognition, i.e., without prior learned representations of the objects to be perceived. This is a fatal flaw if solving AGI is the goal.
Rebel Science, “Deep Learning Is Not Just Inadequate for Solving AGI, It Is Useless” at Medium (Nov 2, 2022)
Yes. Our Amazonian observer would easily see that the bicycle is a metal artifact, a work of craft. Some new observers may think the craft is magic. But at any rate, the bicycle is not a life form or a natural phenomenon.
Some may point out that, even though the Amazon Indian in our example above is able to perceive the bicycle, he will not know what a bicycle is for. This is partly true but neither can a deep neural net even after being trained with millions of images of different types of bicycles. Classification is not understanding, not even close.
Rebel Science, “Deep Learning Is Not Just Inadequate for Solving AGI, It Is Useless” at Medium (Nov 2, 2022)
Wait. Even if the Amazonian observer has never seen or heard of a bicycle before, he might very well have a shrewd idea what it is for. It has, after all, two wheels, a seat, steering handles, pedals, and — probably — a basket. The basket is to hold things. The wheels are for mobility, as the simplest test would show. And the rest — it will soon be determined — is for power and guidance. Our observer might be puzzled as to how the bicycle stays upright when in motion — but only at first. Seeing it in action, he will realize that, when the pedals are moving the wheels, the rider can balance the bike.
The deep neural net will presumably still be classifying images at this point.
Rebel Science points to philosopher Hubert Dreyfus (1929–2017) as offering a useful insight about the importance of generalization: “The late existentialist philosopher and hardcore AI critic, Hubert Dreyfus, was fond of saying that “the world is its own model” and that “the best model of the world is the world itself.” It was his way of explaining that creating stored representations for everything was a mistake.”
But that’s just philosophy right? What impact does the question have on the self-driving car industry?
Deep learning’s inability to generalize is the main reason that the autonomous car industry has failed to produce a full self-driving car. They only have themselves to blame because a number of thinkers have written about the problems with DL when it became popular. Some in the AI community insist that DL’s inability to generalize can be circumvented by scaling but this is obviously not true. Scaling is exactly what researchers in the self-driving car sector have been doing. It does not work. The cost and the many long years it would take to accumulate enough data become untenable because corner cases are infinite in number.
Rebel Science, “Deep Learning Is Not Just Inadequate for Solving AGI, It Is Useless” at Medium (Nov 2, 2022)
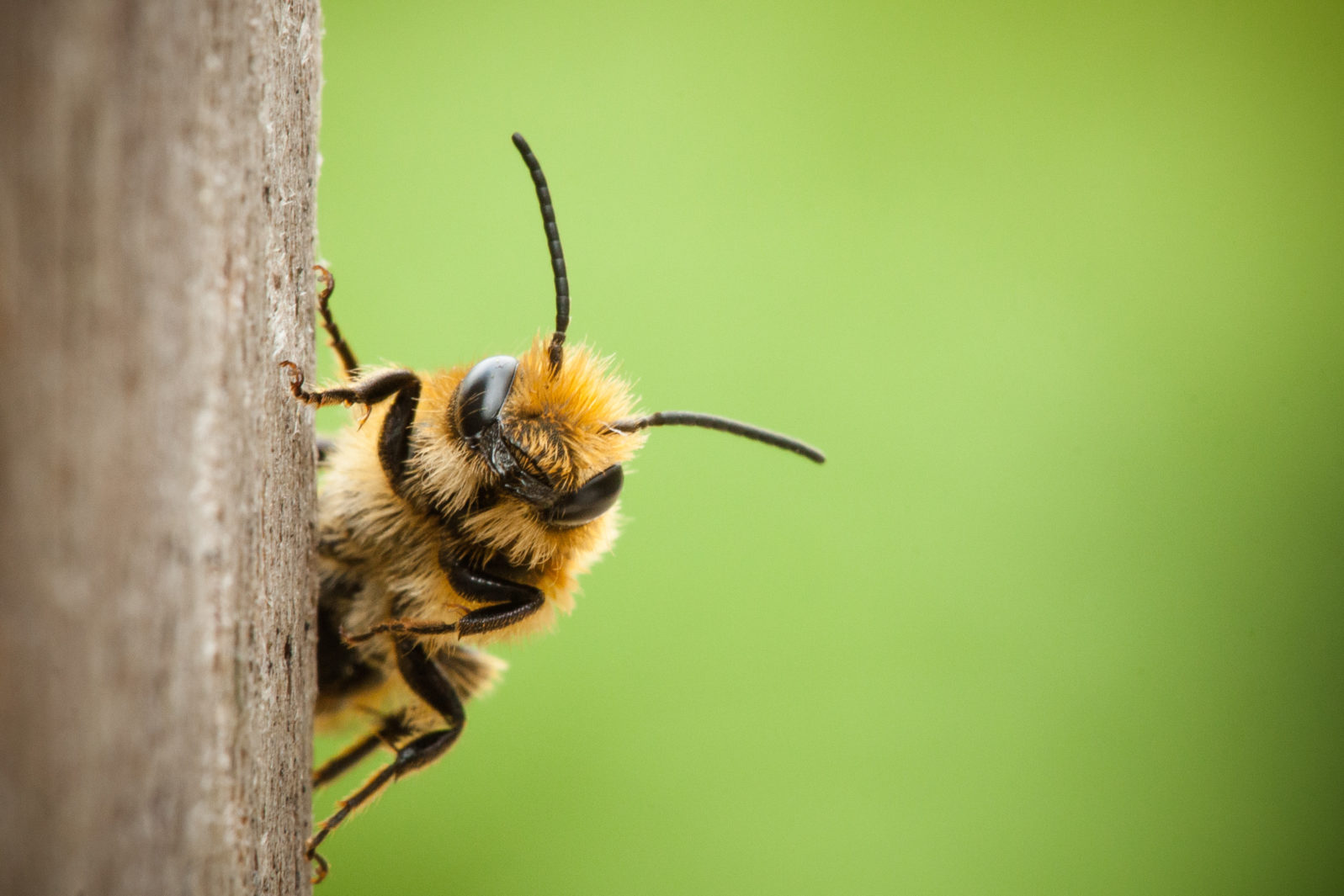
Rebel Science believes that artificial general intelligence is possible in principle, provided the AGI learns to generalize (as the term implies). He differs as to method. He points out, for example, that even bees can generalize; they must do so because their million-neuron brains are too small to store enormous amounts of information. So bigger computer systems, as such, are not the answer. Provocatively, he writes, “By contrast, we believe it is possible to crack AGI on an ordinary desktop computer costing less than $1000.” Provided, of course, the desktop computer can handle generalization.
Rebel Science doesn’t aim for “human-level intelligence” for either self-driving cars or housecleaning robots because he thinks that the cars can get by on 50 million neurons .and the housebots on 100 million. More eclectically, he believes that human-level intelligence could be achieved with less than one billion neurons, as opposed to the 86 billion in the human brain, and that it could be cracked “by a lone maverick working at home.”
He announces, in conclusion, a plan to “release (in the not too distant future) a noise-robust (less than 5 dB SNR) speech recognition demo-application based on our ideas.” Well, we shall see if the new application takes us any closer to generalization.
You may also wish to read: Artificial intelligence: Unseating the inevitability narrative. World-class chess, Go, and Jeopardy-playing programs are impressive, but they prove nothing about whether computers can be made to achieve AGI. In The Myth of Artificial Intelligence, Erik Larson shows that neither science nor philosophy back up the idea of an AI superintelligence taking over. (William Dembski)