AI in Biology: The Disease Connection — When Proteins Go Wrong
Some of the most crucial proteins for human health—the ones we need to understand most urgently—are the very ones that AI has the hardest time modelingThis is the fourth part of Erik J. Larson’s series on the attempt to understand protein folding using AlphaFold. The preceding parts are linked below.
One of the biggest drivers of protein folding research — and of AI-driven efforts like AlphaFold — is drug discovery. The goal is straightforward: understand how disease-related proteins fold and function, then design molecules to target them with precision. But many of the proteins most critical in human disease contain large, disordered regions, making them far harder to model—and far more elusive as drug targets.
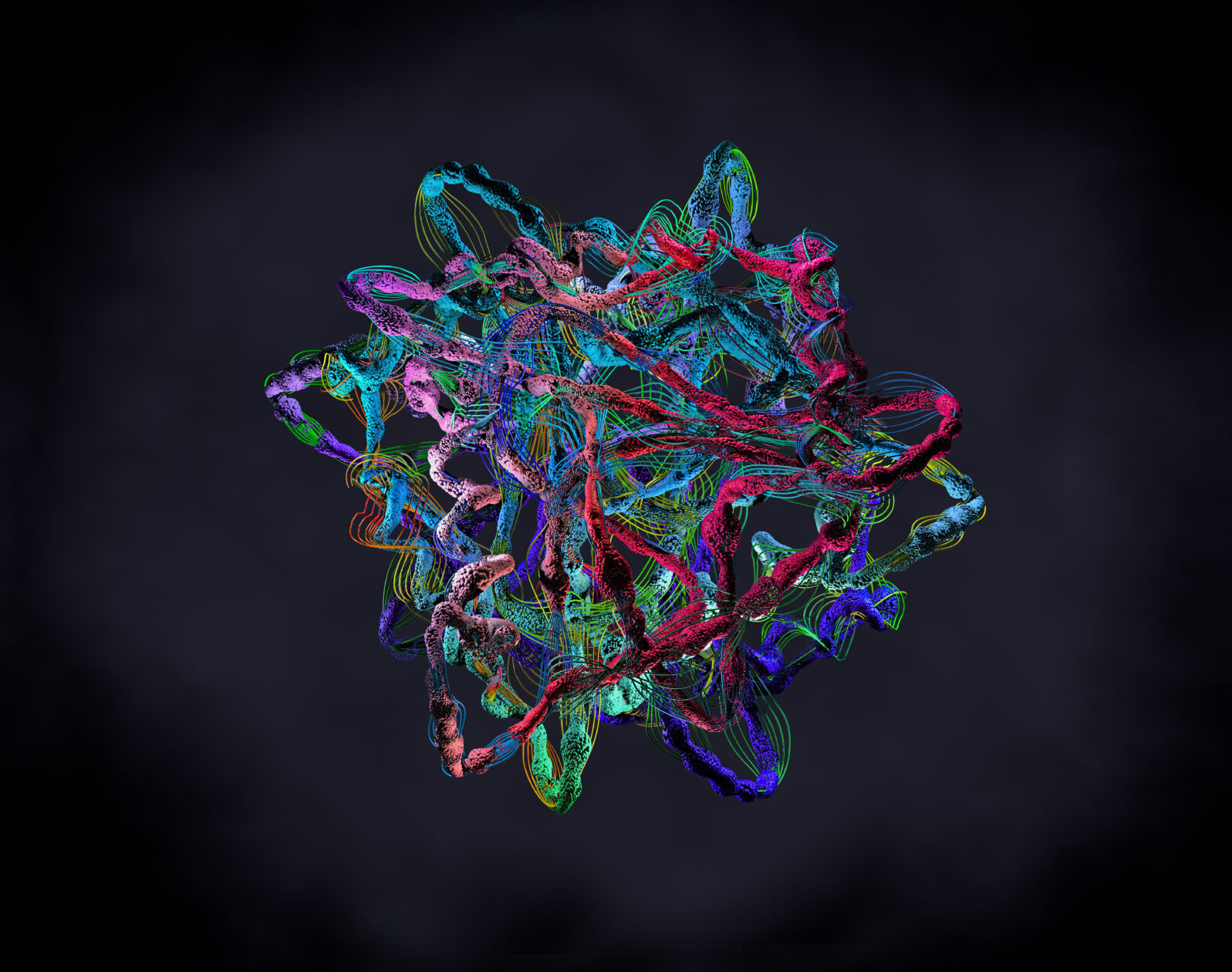
Nowhere is this more evident than in neurodegenerative diseases such as Alzheimer’s and Parkinson’s. IDR-containing proteins like tau and α-synuclein play a central role in both diseases. Under normal conditions, these proteins remain flexible and unstructured — but when they misfold, they clump together into toxic aggregates that destroy brain cells. The transition from functional disorder to lethal aggregation is one of the biggest unanswered questions in molecular biology. And so far, AI has not been up to the task.
Again, it’s not simply that researchers have missed tweaking a button or adding a feature, it’s that the approach itself relies on stable structures. As a result, when disorder is either inherent or is introduced by misfolding, we get low confidence (or no confidence) scores that are, of course, less useful to scientists.
Unfortunately, cancer research faces a similar dilemma. The tumor suppressor p53, often called the “guardian of the genome,” is one of the most studied proteins in biology. Its role? Preventing cells from becoming cancerous. But p53 is not a neatly folded protein — it contains large intrinsically disordered regions that regulate its tumor-fighting activity. When p53 mutates, these disordered regions fail to function, opening the door to uncontrolled cell growth and cancer.
In other words, some of the most crucial proteins for human health—the ones we need to understand most urgently—are the very ones that AI has the hardest time modeling.
Why IDRs defy AI predictions — and traditional computational methods
Intrinsically disordered regions (IDRs) present a fundamental challenge to AI-driven protein structure prediction. Unlike well-folded proteins that adopt a single, stable conformation, IDRs remain structurally heterogeneous. They morph into multiple forms depending on a tangle of factors like cellular conditions, molecular interactions, and biochemical modifications. This variability creates a significant problem for AI models like AlphaFold, which are designed to predict a single “best-fit” structure. The issue is not simply that AI struggles with IDRs—it is that the very premise of IDR behavior contradicts the way these models operate.
To review, one key limitation is the lack of a fixed ground truth structure for IDRs. AI models are trained on structural data obtained from X-ray crystallography and cryo-electron microscopy (cryo-EM), techniques that work best for proteins that crystallize into a well-ordered shape. IDRs, however, do not crystallize and cannot be captured in a single static form. Instead, they exist as a collection of possible conformations, their structure dependent on interactions with other molecules, pH, ionic strength, or post-translational modifications.
Adding to the challenge, IDRs are chemically modified in ways that AI models do not account for dynamically. Post-translational modifications (PTMs) such as phosphorylation, methylation, and ubiquitination can alter an IDR’s shape and function. Yet these modifications are not systematically included in AI training data. Because PTMs are often required for IDRs to assume a biologically relevant conformation, excluding them renders AI-generated predictions incomplete or misleading.
Even when IDRs fold upon interaction with other molecules — such as DNA, RNA, or proteins — their final structure is dictated by the specific biochemical environment, not just by sequence. Predicting their isolated structure, as AI models attempt to do, fails to capture the conditions that determine their function. This isn’t just a flaw — it’s a fundamental crack in the foundation of AI’s “revolutionary” claims.
The computational barriers to IDR prediction
Let’s summarize the three main computational limitations and explain why AI models struggle with IDRs:
- Training Data Bias
Structural databases such as the Protein Data Bank (PDB) primarily contain well-folded proteins that crystallize well, leading to a systematic bias. AI models trained on this data inherit these biases, making them poorly suited for predicting IDRs. - Misguided Assumptions About Protein Folding
AI-driven structure prediction relies on the assumption that proteins seek a single lowest-energy conformation. However, IDRs do not settle into one shape — they function as ensembles of conformations that shift based on their biochemical environment. AI models are not designed to handle this kind of structural variability. - Impact on Drug Discovery
Many disease-associated proteins, including the tumor suppressor p53 (involved in cancer) and tau (linked to Alzheimer’s), contain large IDRs. Since AI-based drug development typically targets stable binding pockets, it is ill-equipped to model IDRs that do not have fixed binding sites. This makes targeting IDRs for therapeutic intervention significantly more difficult.
The TIM-3 Problem: A protein that won’t sit still
The limitations of AI in handling IDRs aren’t just theoretical — they have tangible consequences in real-world drug discovery. Many crucial disease-related proteins resist AI-driven structure prediction precisely because they don’t conform to static, well-folded templates. And no case highlights this more clearly than TIM-3.
If AI models could talk, TIM-3 would be the protein that makes them throw up their hands in frustration. A key player in immune regulation, TIM-3 has been touted as a promising target for cancer immunotherapy. In theory, AlphaFold should be able to predict its structure just as it does for thousands of other proteins. In practice, TIM-3 refuses to cooperate.
But TIM-3 lacks a stable binding pocket, the kind of fixed docking site that makes drug design a straightforward problem of molecular matchmaking. Instead, its shape shifts depending on which molecules it interacts with, adopting different forms under different conditions.
AlphaFold, however, deals in singularities. It wants a best-fit structure, one final answer, when TIM-3 offers nothing but conditional ones. The result? A prediction that looks neat in a database but has little to do with how TIM-3 actually behaves inside a living cell.
Note: Erik J. Larson writes the Substack Colligo.
Here are the first three articles in this series by Erik J. Larson:
AI in biology: AI meets intrinsically disordered proteins. Protein folding — the process by which a protein arrives at its functional shape — is one of the most complex unsolved problems in biology. The mystery of protein folding remains unsolved because, as is so often the case with AI narratives, the reality is much more complicated than the hype.
AI in biology: What difference did the rise of the machines make? AI works very well for proteins that lock into a single configuration, as many do. But intrinsically disordered ones don’t play by those rules. The resulting problems aren’t a temporary bug — they’re a basic limitation of training a machine learning model on a dataset where proteins always fold neatly.
and
AI in biology: So is this the end of the experiment? No. But a continuing challenge is that many of the most biologically important proteins don’t adopt a single stable structure. Their functions depend on structural fluidity. The core issue AI isn’t just missing data — AlphaFold’s entire approach is built on assumptions that don’t apply to disordered proteins.
Here’s the fifth:
AI in biology: The future AI didn’t predict. It doesn’t look like the past. Physical systems that evolve over time but don’t follow a fixed formula have always presented a deep challenge to AI. The problem of outliers or “edge cases” has frustrated AI scientists and engineers (and now structural biologists) for decades, and there’s no good answer yet.