Don’t Worship Math: Numbers Don’t Equal Insight
The unwarranted assumption that investing in stocks is like rolling dice has led to some erroneous conclusions and extraordinarily conservative adviceMy mentor, James Tobin, considered studying mathematics or law as a Harvard undergraduate but later explained that
I studied economics and made it my career for two reasons. The subject was and is intellectually fascinating and challenging, particularly to someone with taste and talent for theoretical reasoning and quantitative analysis. At the same time it offered the hope, as it still does, that improved understanding could better the lot of mankind.
I was an undergraduate math major (at Harvey Mudd, not Harvard) and chose economics for the much the same reasons. Mathematical theories and empirical data can be used to help us understand and improve the world.
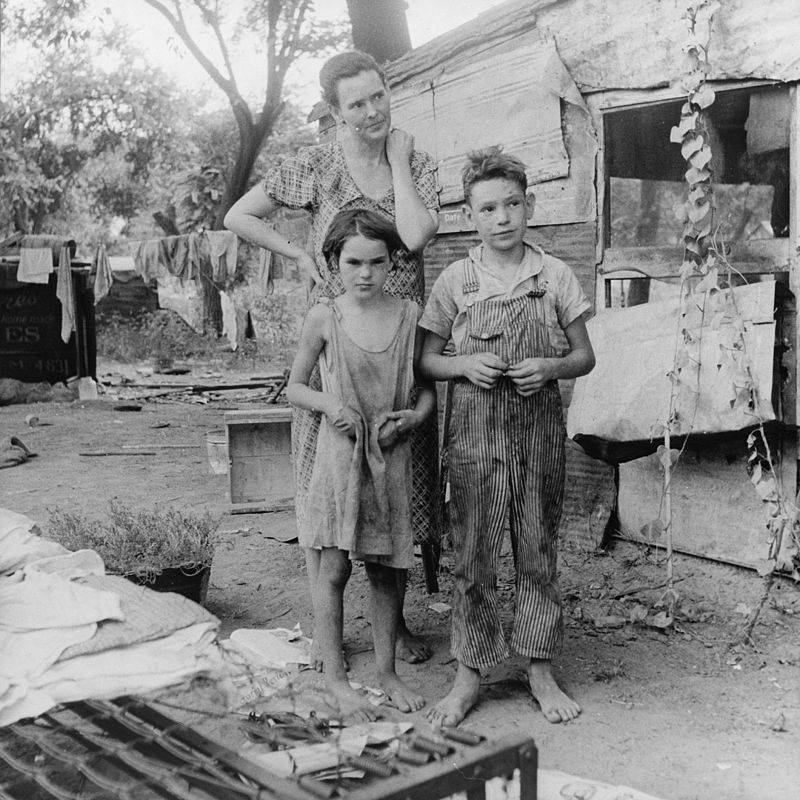
For example, during the Great Depression in the 1930s, governments everywhere had so little understanding of the economy that their policies were utterly counterproductive. They cut spending, raised taxes, allowed the money supply to shrink, and engaged in trade wars. Today, thanks to theoretical and empirical economic models, we (well, most of us) know that these are exactly the wrong policies for fighting economic recessions.
This is why, during the global economic crisis that began in the United States in 2007, governments did not repeat the errors of the 1930s. With the world economy on the brink of a second Great Depression, governments did the right thing by pumping trillions of dollars into their deflating economies.
On the other hand, economists can be seduced into constructing models that are mathematically elegant but utterly unrealistic. When I was an assistant professor at Yale, a future Nobel laureate began a seminar by announcing flippantly that he had made “whatever assumptions are needed.”
At another seminar, a prominent economist started his talk by specifying two assumptions that he needed in order to prove several theorems about economic behavior. As he worked his way through the math, the Nobel laureate sitting next to me was scribbling furiously on a notepad. I thought he was checking the math. But when the speaker finished and asked for questions, the Nobel laureate said, “I want to talk about your second assumption.” That is exactly right. The validity of the assumptions is often more important than beauty of the math. “Garbage in, garbage out” applies not only to data, but to theories.
The great British economist John Maynard Keynes (1883–1946) wrote that
The master-economist must possess a rare combination of gifts. He must be mathematician, historian, statesman, philosopher in some degree. He must understand symbols and speak in words. He must contemplate the particular in terms of the general, and touch abstract and concrete in the same flight of thought. He must study the present in the light of the past for the purposes of the future. No part of man’s nature or his institutions must lie entirely outside his regard.
Tobin was a master economist (and a great Keynesian). He knew when math could be useful and enlightening — and he also knew that economics is ultimately about people, not math.
I have been thinking about this while writing a book about investing. Mathematical convenience has often trumped common sense in financial models. For example, it is often assumed — because the assumption is useful — that changes in stock prices can be modeled as independent draws from a probability distribution. Paul Samuelson offered this analogy:
Write down those 1,800 percentage changes in monthly stock prices on as many slips of paper. Put them in a big hat. Shake vigorously. Then draw at random a new couple of thousand tickets, each time replacing the last draw and shaking vigorously. That way we can generate new realistically representative possible histories of future equity markets.
I did Samuelson’s experiment. I put 100 years of monthly returns for the S&P 500 in a computer “hat” and had the computer randomly select monthly returns (with replacement) until I had a possible 25-year history. I repeated the experiment one million times, giving one million “Samuelson simulations.”
I also looked at every possible starting month in the historical data and determined the very worst and very best actual 25-year investment periods. The worst period began in September 1929, at the start of the Great Crash. An investment over the next 25 years would have had an annual return of 5.1%. The best possible starting month was January 1975, after the 1973-1974 crash. The annual rate of return over the next 25 years was 17.3%.
In the one million Samuelson simulations, 9.6% of the simulations gave 25-year returns that were worse than any 25-year period in the historical data and 4.9% of the simulations gave 25-year returns that were better than any actual 25-year historical period. Overall, 14.5% of the Samuelson simulations gave 25-year returns that were too extreme. Over a 50-year horizon, 24.5% of the Samuelson simulations gave 50-year returns that were more extreme than anything that has ever been experienced.
Why do the Samuelson simulations give such unrealistically extreme outcomes?
In the real world, long-run movements in stock prices are tied (admittedly loosely) to fundamentals. They cannot randomly walk to permanently excessive lows or highs. You can lose all your money betting on dice rolls because these are independent events. The S&P 500 will not go to zero. Stock prices will eventually stabilize and rebound because stocks will become temptingly cheap if earnings and dividends rise and stock prices don’t. For similar reasons, stock market bubbles do not last forever. Unlike dice rolls, stock prices are anchored by fundamentals.
The unwarranted assumption that investing in stocks is like rolling dice has led to some erroneous conclusions and extraordinarily conservative advice. For example, after the 2007 stock market crash, Zvi Bodie, a prominent economist and financial advisor, sounded apocalyptic:
Prices dropped by 37% last year. While improbable, there’s nothing to say they couldn’t drop by that much again next year or the year before you retire. And diversification doesn’t take away that risk. That’s why retirement money belongs in truly safe assets whose value won’t go down not in stocks.
A 37% price drop might be drawn over and over in a Samuelson simulation but won’t happen in the real world. At some point, stock prices will be so low relative to corporate earnings and dividends that investors will find stocks irresistible and stock prices will stop free falling.
With exquisitely bad timing, in March of 2009, Bodie advised people to sell all their stocks:
Unless you have the heart of a high stakes gambler, get out of stocks now and put your retirement money in inflation protected government bonds and similar instruments. These investments are immune to the kind of calamity Wall Street experienced last year.
When an interviewer asked Bodie, “Wouldn’t leaving the stock market right now be locking in your losses?,” Bodie replied, with no evident irony, “That is exactly right. You want to make sure you don’t lose more.”
The stock market bottomed on March 9, 2009, and then surged upward:
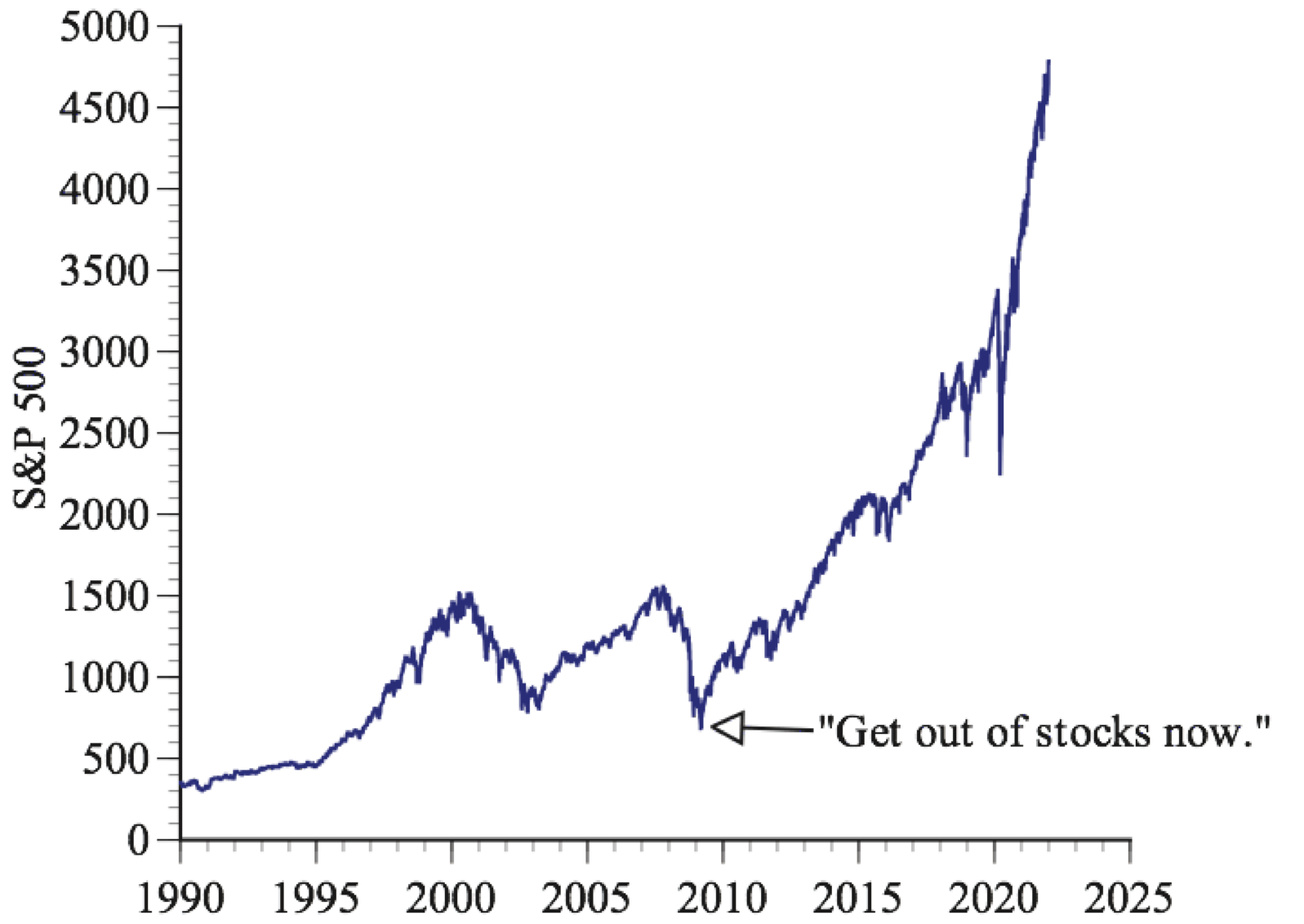
The point is not that Bodie was spectacularly wrong (we’ve all made mistakes), but that it is hazardous to your wealth to think that the stock market is like a sequence of dice rolls. More generally, the proper test of an economic model is not whether the math is impressive, but whether it improves our understanding of the world.
You may also wish to read: The stock market keeps rising despite COVID. Is it nuts? I’ve been asked whether advanced AI can explain the conundrum. AI can’t, by itself, predict or explain stock trends. But a look at profitability might help. (Gary Smith)