The AI Hype Machine Just Rolls On, Living on Exhaust
Even chatbot enthusiasts, are starting to admit that scaling up LLMs will not create genuine artificial intelligenceArtificial intelligence (AI) enthusiasts have had a long and inglorious history of overpromising and underdelivering. Nearly sixty years ago, in 1965, Economics Nobel Laureate and Turing Award winner Herbert Simon (1916–2001) made the confident prediction that “machines will be capable, within 20 years, of doing any work a man can do.” Five years later, Turing Award winner Marvin Minsky (1927–2016) said that, “in from three to eight years we will have a machine with the general intelligence of an average human being.” As the years passed, the failed predictions were replaced by new, equally overly optimistic, predictions.
One thing we have learned from the many decades of very smart people trying to build computers that are as intelligent as they are is how truly remarkable our brains are—and how little we understand about how they work. Human brains are not just pattern seekers and curve fitters. They are not just computers.
For example, an image-recognition computer program might train on thousands or millions of pictures of children’s wagons, looking for pixel patterns that it can associate with the label “wagon.” When shown a new picture of a wagon, the program might generate the wrong label because the wagon is made of a different material, painted a different color, viewed from a different angle, or partly obscured.
Children, on the other hand, need only see a handful of wagons to understand the essential structure: a box with four wheels and a handle. They know it is a wagon regardless of whether it is made our metal, wood, or plastic; regardless of whether it painted red, green, or candy-striped. If they see two wheels on one side, they expect to see two wheels on the other side.
When an adult and child approach a wagon, they are not surprised if the child climbs inside and is pulled by the adult. They are amused if the child pulls the adult. If they want to move a dozen bricks from one place to another, they know it will be easier if the bricks are put in a wagon and pulled, even if they have never seen bricks in a wagon before. If a wagon is at the top of a hill, they know it might be exciting and dangerous to sit in the wagon, even if they have never seen a wagon roll downhill.
I could go on. My point is simply that there is a qualitative difference between finding statistical patterns in numbers or pixels and making sense of the world we live in.
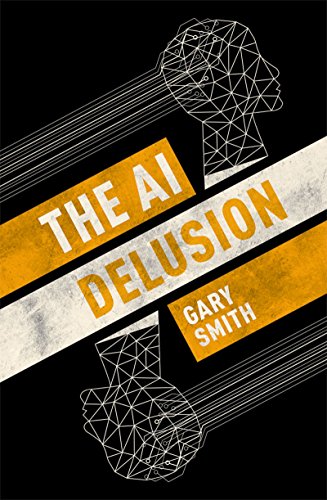
When I wrote The AI Delusion in 2018, my elevator pitch was that the real problem is not that computers are smarter than us but that we think computers are smarter than us and consequently trust them to make decisions they should not be trusted to make. Among the examples were Google’s failed attempt to predict flu activity based on Google searches, a program that evaluated job applicants based on the websites they visited, a program that assessed loan applicants based on their smartphone usage, a program that based car insurance rates on the words applicants used in their Facebook posts, and black box models for predicting criminal behavior.
Recently, the AI hype machine has been fueled by the text-generating prowess of ChatGPT, Copilot, Gemini, and other large language models (LLMs). Alas, these models’ confident glibness masks the reality that they do not know what words mean or how words relate to the real world, so they cannot assess the veracity of the text they generate, create a causal understanding of events, or employ critical thinking skills. Here is an example.
Yet, it is hard to quiet the hype, particularly when fake-it-til-you-make-it schemes can attract so many customers and so much funding. The AI-startup bubble may come to rival the dot-com bubble and end just as badly.A few days ago, during an X Spaces podcast interview, Elon Musk asserted that, “AI is the fastest advancing technology that I’ve ever seen of any kind, and I’ve seen a lot of technology … My guess is that we’ll have AI smarter than any one human around the end of next year.”
His evidence was a graph showing that computational capacity had increased exponentially between 2011 and 2021: “Many, many software breakthroughs are demonstrated on the curve.” But the curve does not, in fact, demonstrate any software breakthroughs. It only shows increases in computational capacity, which has little to do with genuine intelligence.
It is increasingly accepted, even by LLM enthusiasts, that scaling up LLMs will not get us to artificial general intelligence (AGI)—the ability to perform any intellectual task that human beings can do. For instance, on January 16 on this year, Yann LeCun Chief AI Scientist at Meta, said that, “The future of AI is not generative. It will not get us to where we need to go.”
A specific example is that LLMs have been training on larger and larger databases but in a forthcoming article in the Journal of Financial Planning, I show that they still cannot answer basic financial questions that require critical thinking. The solution is not for LLMs to ingest more words but for humans to find ways for computers to truly understand words. We are still far from that goal.
Of course, Elon Musk has been overly optimistic in the past. In 2016, he said that “all Tesla vehicles exiting the factory have the hardware necessary for Level 5 autonomy. Every car we make, on the order of 2,000 cars a week, are shipping now with Level 5, meaning hardware capable of full self-driving, or driverless, capability.” As the years have passed, Level 5 continues to be predicted but is not close to being realized.
Perhaps because of skepticism about LLMs or perhaps because of skepticism about Musk’s cheerleading, Damion Hänkejh, CEO of ingk.com, offered to bet Musk $10 million that he is wrong about AI being smarter than any human by the end of 2025. Or perhaps Hänkejh welcomed the publicity.
In any case, I expect that it will be difficult to settle on iron-clad rules for such a wager. If a bet is agreed upon, the only people who are likely to profit will be the lawyers arguing about who won.
Meanwhile, the AI hype machine will continue, largely disconnected from reality.