The Threat That Deepfakes Pose to Science Journals
Image manipulation has been a problem for decades but convincing deepfakes could magnify the problem considerablyWhen a team of researchers at Xiamen University decided to create and test deepfakes of conventional types of images in science journals, they came up with a sobering surprise. Their deepfakes were easy to create and hard to detect.
Generating fake photographs in this way, the researchers suggest, could allow miscreants to publish research papers without doing any real research.
Bob Yirka, “Computer scientists suggest research integrity could be at risk due to AI generated imagery” at Tech Explore (May 25, 2022)
They created the deepfakes in a conventional way by starting with a competition between two powerful computer systems:
To demonstrate the ease with which fake research imagery could be generated, the researchers generated some of their own using a generative adversarial network (GAN), in which two systems, one a generator, the other a discriminator, attempt to outcompete one another in creating a desired image. Prior research has shown that the approach can be used to create images of strikingly realistic human faces. In their work, the researchers generated two types of images. The first kind were of a western blot—an imaging approach used for detecting proteins in a blood sample. The second was of esophageal cancer images.
Bob Yirka, “Computer scientists suggest research integrity could be at risk due to AI generated imagery” at Tech Explore (May 25, 2022)
Here are the deepfakes they created:
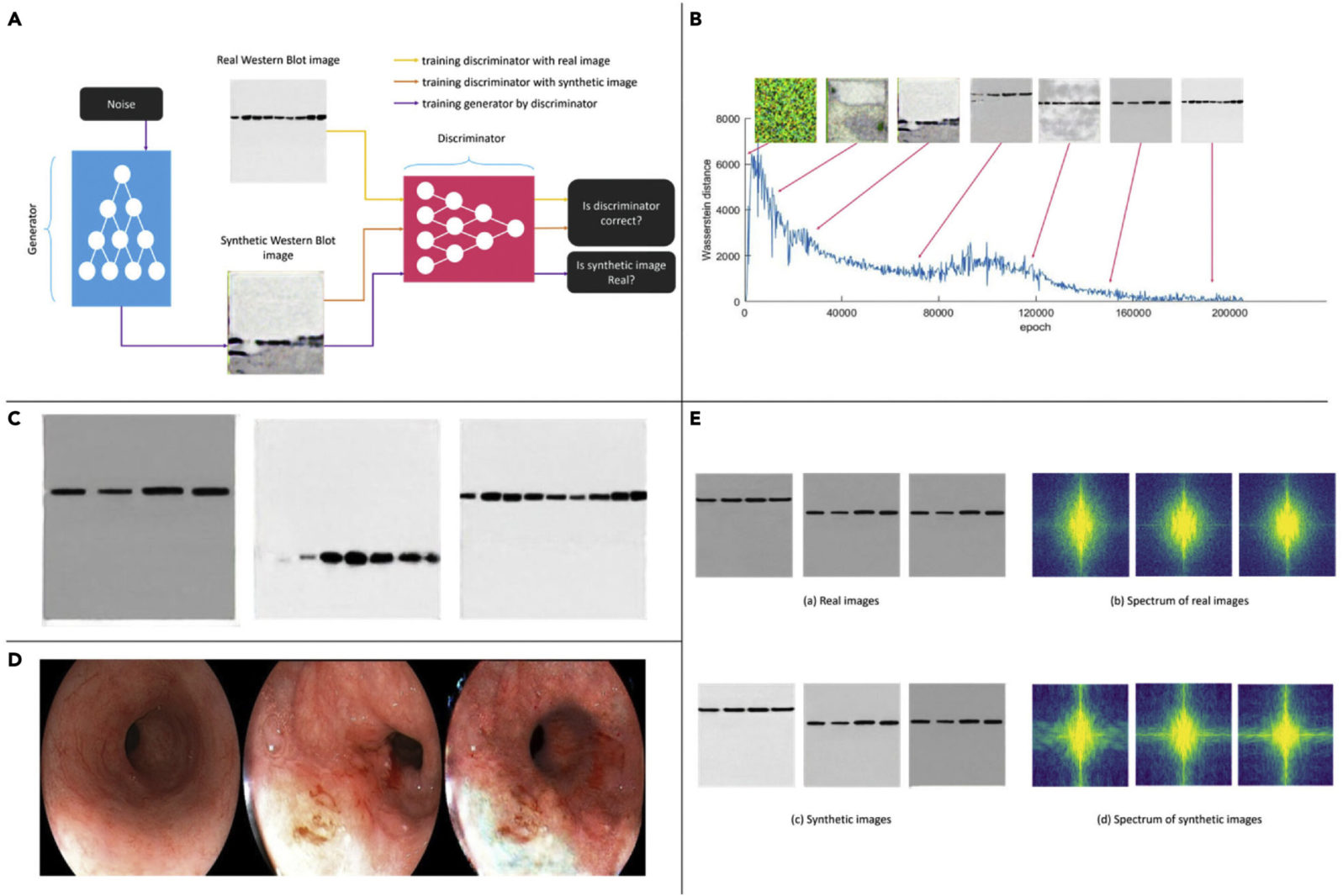
How difficult is it to detect deepfakes? The researchers proceeded to try out some of their fake images on experts in the field:
It is very difficult, if not impossible, for the naked eye to distinguish between real and fake images generated by AI. In a subjective quality-evaluation test, we invited three board-certified biomedical specialists to evaluate the quality of the generated western blot images. A series of image groups were displayed to the subjects, where each group contained three real images and a fake one in random order unknown to the subjects. The subjects were then asked to identify the fake one. Among them, two experts achieved accuracy levels of 10% and 30% respectively, suggesting that they did not perform better than random guessing in identifying the fake western blot images generated from GAN. The third expert achieved an accuracy of 60% based on a subtle difference between real and fake western blot images; i.e., the boundary between synthetic blots and the background is not as smooth as that between real blots and the background. This is introduced by the intrinsic properties of GAN-based methods and can be dealt with by further optimizing the network model.
Liansheng Wang, Lianyu Zhou, Wenxian Yang, Rongshan Yu, Deepfakes: A new threat to image fabrication in scientific publications?, Patterns, Volume 3, Issue 5, 2022, 100509, ISSN 2666-3899, https://doi.org/10.1016/j.patter.2022.100509. The paper is open access.
Just now, it is not clear what to do about the problem. Advances in deepfake detection will be met by advances in deepfake creation.
Perhaps professional associations must take the lead in getting all new images scanned by the most advanced deepfake detectors, with dire consequences on discovery. In that case, only those using the most sophisticated equipment would risk trying. But even then, if tests are routinely rerun later — on a randomized basis — with even more advanced deepfake detection equipment, deepfakers would face the ongoing risk of future career ruin, even if they escape immediate consequences. It would be somewhat like lying about one’s qualifications on one’s resume. Such a falsehood can lead to termination even if it is only discovered years later.
Addressing the problem may come down to how seriously science journals choose to take deepfakes. We were told at Nature in 2009 that journals were cracking down on image manipulation of various types (not necessarily deepfakes). But in 2016, Elizabeth Bik et al. found that the problem was still prevalent in biomedical research:
Bik assigned problematic images to five categories: simple duplication, duplication with repositioning, duplication with alteration, cuts, and beautification.
Cuts and beautification (the latter of which can assist readers afflicted by color blindness) don’t always constitute research misconduct. Duplication almost always does. Whether that misconduct is intentional or accidental, the experiments based on flawed findings are invalid and papers citing manipulated images must be retracted. That’s why the majority of the scientific community agrees that journal editors or peer reviewers have an important role in identifying data integrity issues before publication.
Yet even after the Hwang and Yin scandals, many such violations of image integrity are discovered by readers after publication, partly because discerning unethical image processing can be very difficult. As one PubPeer commenter notes, “It is so easy to cheat … without leaving traces …”
Enago Academy, “Scientific Fraud: How Journals Detect Image Manipulation (Part 1)” at Enago (July 20, 2019)
Then, in 2020, major science publisher Elsevier admitted that “Image manipulation is still a serious issue in scholarship”. Deepfakes have, however, greatly raised the stakes for the journals.
Here’s a video on how much more quick and simple deepfakes have become:
You may also wish to read: Deepfakes can replicate human voices now — maybe yours. Digitally faked voice tech has already been used to perpetrate a big bank fraud, Even as deepfakes grow more sophisticated, the technology to detect them lags behind the technology to create them.