Could Machine Learning Decipher Lost Languages?
It gives us new search powers, based on perennial facts of languageLanguages get lost when no one still alive knows them and there is no written record. Or they can get lost when there is a written record but no one alive can read it. To see what machine learning can and can’t do, let’s look at the historic case of a long-sought lost language, Minoan A.
In 1886, British archaeologist Arthur Evans found a number of stones and tablets written in two different scripts on the Mediterranean island of Crete. One, called Linear B, was dated from about 3500 years ago (1400 BCE) when Mycenaean Greeks ruled the island. The other, Linear A, is even older. It dates back to the period between 1800 and 1400 BCE, when a Bronze Age civilization, the Minoans, ruled.
Linear B was decoded in 1953. No one has ever decoded Linear A. Could machine learning help?
First, let’s look at how Linear B was decoded. In 1953, cryptographer Michael Ventris (1922–1956) succeeded, via a key intuition about language followed up by an educated guess.
First, languages don’t accidentally have meaning; they inherently have meaning. And the meaning they have is related to what human beings think about. Most specifics of language flow from what matters to us. Second, Ventris guessed that the language was related to at least one other language in which documents have survived:
His solution was built on two decisive breakthroughs. First, Ventris conjectured that many of the repeated words in the Linear B vocabulary were names of places on the island of Crete. That turned out to be correct.
His second breakthrough was to assume that the writing recorded an early form of ancient Greek. That insight immediately allowed him to decipher the rest of the language. In the process, Ventris showed that ancient Greek first appeared in written form many centuries earlier than previously thought.
Emerging Technology from the arXiv, “Machine learning has been used to automatically translate long-lost languages” at Technology Review
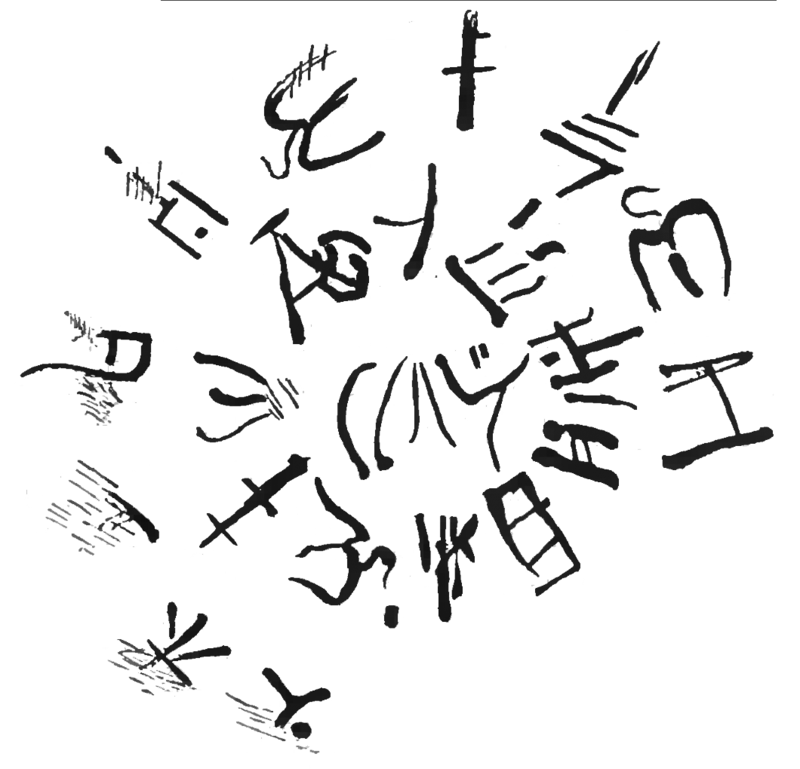
Unfortunately, that approach wouldn’t help with Linear A, described starkly at Technology Review as “one of the great outstanding problems in linguistics to this day,” because those methods haven’t worked.
Might machine learning help? It’s not just the huge amount of data that a machine can motor through. Rather,
The big idea behind machine translation is the understanding that words are related to each other in similar ways, regardless of the language involved.
So the process begins by mapping out these relations for a specific language. This requires huge databases of text. A machine then searches this text to see how often each word appears next to every other word. This pattern of appearances is a unique signature that defines the word in a multidimensional parameter space. Indeed, the word can be thought of as a vector within this space. And this vector acts as a powerful constraint on how the word can appear in any translation the machine comes up with.
Emerging Technology from the arXiv, “Machine learning has been used to automatically translate long-lost languages” at Technology Review
That’s not a new idea, of course. An endless variety of word games derives from the fact that only certain combinations and orderings of words can be correct. Machine learning leverages this fact just as Ventris did, but with vastly more resources.
Such resources might help with lost languages. A lost language, for example, may turn out to be a descendant of another language, in which case the changes over time usually follow predictable patterns. That is, if speakers of the language sounded “s” as “sh,” they probably did that with most words beginning with “s.” With only a bit of new evidence, it might be possible to work backward, using an earlier language that used only “s.”
Jiaming Luo and Regina Barzilay from MIT and Yuan Cao from Google’s AI lab in Mountain View, California. re-deciphered Linear B to show that Linear A might, in principle, be cracked by machine learning.
But that’s all they could do. Linear A does not seem to be a form of Greek and no one knows what language it is a form of. So comparative language techniques, needed for machine learning as well as other methods, can’t be used.
If we had any idea what the Linear A people were talking about (a war? a marriage? a contest? taxes? the gods?), we might begin to develop a clue. Maybe archeology will help someday. For example, if characters keep appearing on tokens, they probably mean something official. At least we know the writers were human beings and even a single connection will help.
Sometimes that’s all you have to go on.
The origin of language remains obscure One problem is that information is not measured in science in a way that relates to matter and energy. (Denyse O’Leary)
The real reason why only human beings speak (Michael Egnor) Language is a tool for abstract thinking—a necessary tool for abstraction—and humans are the only animals who think abstractly
and
How is human language different from animal signals?