Why Humans Aren’t That Biased, and Machines Aren’t That Smart
Claims about the cognitive biases that supposedly overwhelm our judgment should be taken with a helping of saltThis article first appeared at Colligo and is reprinted here with permission.
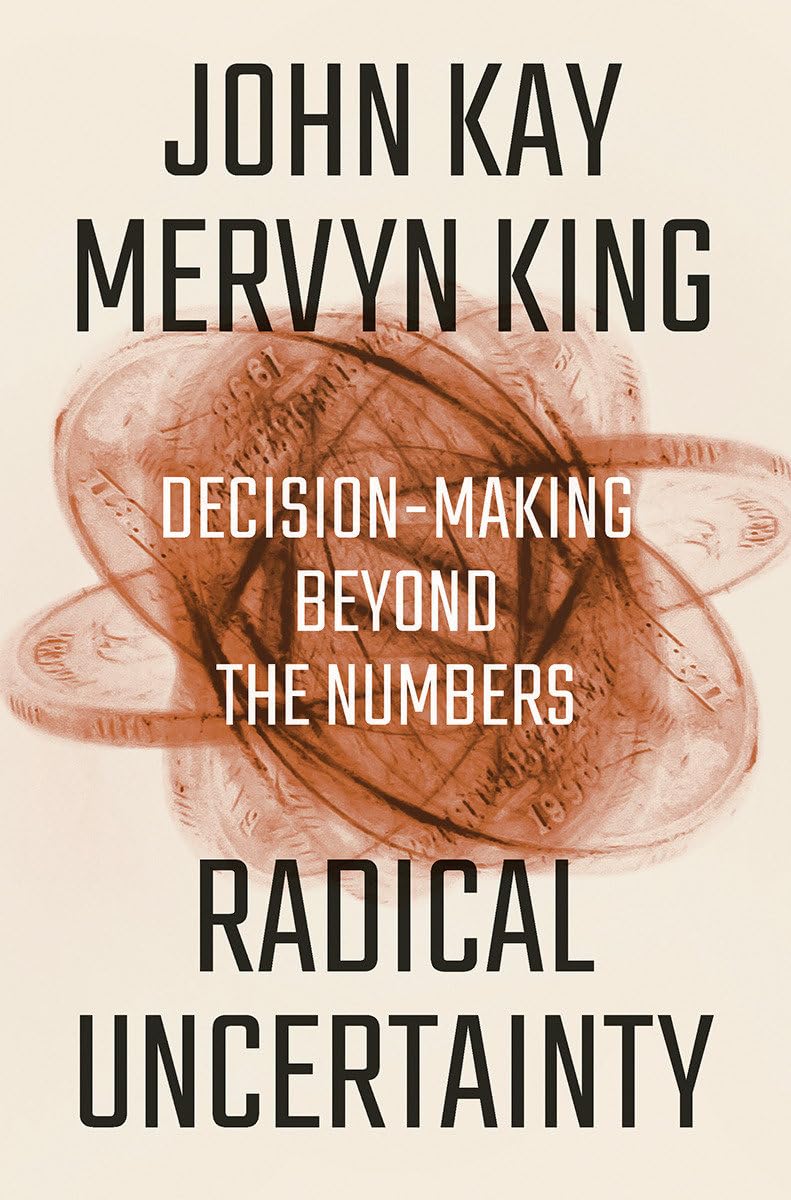
Later this month, I’ll be on a panel in Savannah, Georgia, for a Liberty Fund colloquium on “AI and Technosocialism.” This will be my second time participating — my first was nearly twenty years ago at a scenic retreat outside Tucson, Arizona. This year’s discussion centers on an intriguing book: Radical Uncertainty: Decision-Making Beyond the Numbers (Norton 2020) by economists John Kay and Mervyn King. Their focus on how we make judgments under “radical uncertainty” has important implications for AI, which is why I’m writing this post.
First, let’s look at the experts and their own biases — particularly in how they label human reasoning as “cognitive bias.” An obsession with the supposed precision of math and computation has left multiple fields, like economics, psychology, and cognitive science with reams of research purportedly establishing our “natural stupidity.”
Not so. Read on.
Why cognitive bias is biased
I first encountered the “Linda Problem” in a class on epistemology, part of the philosophy department’s curriculum. The “Linda problem” is one of a number of arresting examples of human stupidity offered over the decades by the late Nobel economist Daniel Kahneman (1934-2024) and his partner Amos Tversky (1937–1006), both Israeli psychologists working in America. From the 1970s up to the 21st century, Kahneman and Tversky left such a large footprint on thinking about thinking that you’re likely to encounter them not just in philosophy but in economics, psychology, and even classes on math and statistics.
The two went mainstream in culture too, notably due to the efforts of writer Michael Lewis, who wrote Moneyball and The Big Short (both were turned into major motion pictures). He also brought Kahneman and Tversky to bookshelves with his 2016 The Undoing Project.
”Undoing” here is the idea that humans think rationally
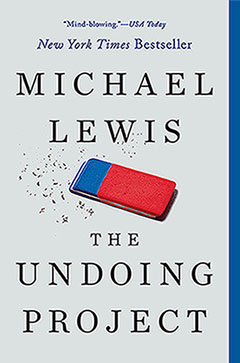
The “undoing project” is an apt description of what Kahneman and Tversky were up to. What they were intent on undoing here is the idea that humans think rationally and aren’t generally plagued by cognitive bias. Au contraire, according to K & T we don’t think rationally even when we think we do, and our mental shortcuts amount to distortions — cognitive bias — that we later rewrite to fit the facts, rather than face our own “natural stupidity.”
I haven’t read The Undoing Project, but knowing Lewis’s work, I suspect it’s a compelling narrative. I also have no doubt that K & T didn’t accomplish what they’d hoped — we’re not hopelessly biased and irrational in the way they meant. The problem here is the presupposition of axiomatic rationality. That is appropriate for problems like games or more generally what economists call “small world” problems with known rules and constraints, and a well-defined optimal outcome. Think of poker, where there’s no ambiguity about the best possible hand.
Back to the Linda problem. In 1983, Kahneman and Tversky published in the journal Psychological Review a paper titled “Extensional versus Intuitive Reasoning: The Conjunction Fallacy in Probability Judgment.” In the paper, K & T reported their study where they presented participants with the following scenario1:
Linda is 31 years old, single, outspoken, and very bright. She majored in philosophy. As a student, she was deeply concerned with issues of discrimination and social justice and also participated in anti-nuclear demonstrations.
Which of the following is more likely?
Linda is a bank teller.
Linda is a bank teller and is active in the feminist movement.
The most common answer (given by 85% to 90% of college undergraduates at major universities) was Option 2. This is wrong according to probability theory, since the probability of two events A and B occurring together cannot exceed the probability of either event occurring alone. K & T were surprised to discover that the error persisted among the majority of the participants even when it was pointed out.
Assuming that “axiomatic rationality” requires adherence to the laws of probability, they concluded that people have a “conjunctive bias.” The conjunctive bias result then leads to entire classes of biases that have now been broadly absorbed into society, for example:
Probability & Statistical Biases
● Conjunction Fallacy – Assuming that specific conditions are more likely than a single general one (e.g., the Linda Problem).
● Base Rate Neglect – Ignoring general probability rates in favor of specific details.
● Gambler’s Fallacy – Believing past events affect the likelihood of independent future events (e.g., “I’ve lost five times, so I must win the next round”).
Risk & Loss Perception Biases
● Loss Aversion – Feeling the pain of losses more strongly than the pleasure of equivalent gains.
● Optimism Bias – Believing that negative events are less likely to happen to oneself than to others.
● Negativity Bias – Giving more importance to negative experiences than positive ones.
Decision-Making & Belief Biases
● Confirmation Bias – Tendency to search for, interpret, and remember information that confirms pre-existing beliefs.
● Availability Heuristic – Overestimating the importance of information that is most readily available (e.g., fearing plane crashes over car accidents due to media coverage).
● Anchoring Bias – Relying too heavily on the first piece of information encountered (the “anchor”) when making decisions.
● Overconfidence Bias – Overestimating one’s knowledge, ability, or predictions.
● Hindsight Bias – Believing, after an event has occurred, that it was predictable (“I knew it all along”).
● Framing Effect – Making different decisions based on how the same information is presented (e.g., “90% survival rate” vs. “10% mortality rate”).
Memory & Perception Biases
● Peak-End Rule – Judging an experience based on its peak (most intense moment) and its end rather than the total experience.
● Recency Bias – Giving more weight to recent events than earlier ones.
● Serial Position Effect – Remembering the first and last items in a list better than the middle ones.
Cataloguing these ostensible biases certainly has some value — presumably we would want hapless gamblers to internalize the idea of sunk cost fallacies. But the broader point here is negative: Kahneman and Tversky, and cognitive biases themselves, assume a picture of rationality—called, again, “axiomatic rationality” as it’s derived from math and statistics — that works only when we know the rules of the game, and we can determine, perhaps with more information, the outcomes. Probability theory, a relatively modern concept in the history of thought, traces back to 1654 when a French gambler, Chevalier de Méré, sought help from Blaise Pascal (1623–1662) in resolving a paradox in a popular dice game.
The details would take us too far afield, but it suffices to say that de Méré’s confusion anticipated several centuries of more recent confusion about the role of models as applied to the real world. Pascal was able to (quite brilliantly) resolve de Mere’s complaint that his expectations of winning didn’t match reality. But he succeeded because he had a small world to consider, rather than the large world we inhabit outside of card games and gambling.
Still, the notion that we can reduce the real world to a card game, in effect, and compute probabilities for outcomes seems an almost irresistible charm for modern researchers hoping to look clever by application of probability and math to big picture problems in social, psychological, environmental issues, and other contexts. Indeed, Kahneman and Tversky and others of their ilk have convinced generations of scholars, students, and the rest of us that we’re walking dunderheads. Let’s go back to the Linda problem and see why they — not we — are wrong.
The Linda Problem revisited
The resolution to the Linda Problem is not that 90% of the educated population exhibits the “conjunctive bias,” but rather that most people — 90%! — interpret the description as a story rather than a math problem. They are thinking about likelihood given the information given, not about axiomatic rationality and probability theory. So, why should they trot out the “law” of conjunction in probabilities? Likelihood is more about what we expect to see and much less about the outcome of a calculation. As Kay and King put it:
The subjects were not asked about probabilities but about likelihood, and they answered the examination question Kahneman asked rather than the one he thought he was asking.
Kay and King’s analysis is spot on, as far as I’m concerned, and we can add an additional bit of evidence that they didn’t discuss, known in linguistics and philosophy as “Grice’s maxims,” summed up by the cooperation principle:
Make your contribution as informative as is required (for the current purposes of the exchange) but not more so.
Applied to the Linda Problem, Grice’s cooperation principle for communication implies that there was some point to offering the hearer the information that Linda was active in issues of discrimination and social justice. Readers then naturally expect this additional description to enter into their judgement of what’s likely, or not likely, about her. Given that the two options differ in precisely the way the additional information is framed, it’s natural for participants to interpret the second as the more complete and plausible description of Linda’s life. If no one is thinking about doing calculations — and participants were not instructed to do so — it’s hardly fair to tag them with a “conjunctive bias.”
Similar remarks can be made about many of the other biases, which also assume some implausible standard like “axiomatic rationality” when presented with “big world” or in other words realistic or “real world” problems. In such cases, people not only don’t rely on formal mathematical reasoning but can’t rely on it, since the problems can’t be reduced to a set of rules or principles with known or discoverable outcomes. So much for four decades of bias and the conclusion that humans are naturally stupid.
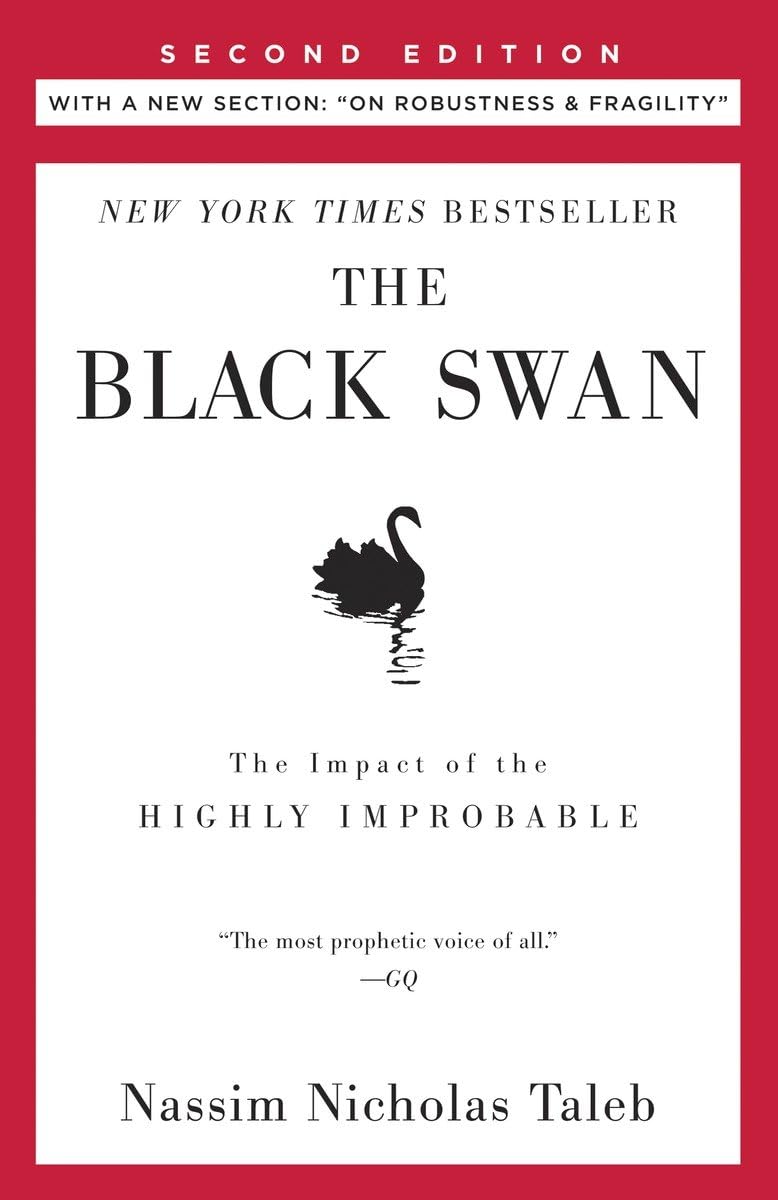
To be fair, research on cognitive bias can illuminate how we think and make judgements. But by assuming a standard like axiomatic rationality, researchers on bias tend to miss the real reasons we use heuristics or short cuts rather than do calculations in the real or “large world” with lots of “unk-unks,” or “unknown unknowns.”
Kay and King call our “muddling through” the world of radical uncertainty evolutionary or ecological rationality. Ecological rationality is well-suited to our real world of radical uncertainty, where outcomes are either not envisioned at all — Nassim Nicholas Taleb ’s “black swans” — or we understand the range of possible outcomes but additional ratiocination or data gathering won’t resolve what happens. For instance, loss aversion is a bias in Kahneman and Tversky’s world, but it’s pretty useful if you’re crossing a busy street, or walking around an African savannah. As Kay and King put it, “A disposition to avoid large losses is a useful attribute.”
Not always.
When risk tolerance is useful
In business and sports, risk tolerance if useful as well. Indeed, insight and creativity aren’t part of axiomatic rationality and can’t be, since by nature they can’t be predicted. It would seem to follow that examples of insight and creativity are, by the standards or axiomatic rationality, weirdly irrational: on what basis did you believe you could “bend reality to your will, Mr. Jobs?”
In fact, a good case can be made that entrepreneurship and capitalism in the vein of Adam Smith (1723–1790) is based on a bunch of cognitive biases. Business icons like Steve Jobs (1955–2011) or Richard Branson are known to have taken huge risks, not only in pursuit of business dreams but in their personal life.
Branson reportedly started his business empire by making huge bets at a gambling table. Such a move, assessed in terms of probabilities, is foolish more often than not.
But the broader point, as Kay and King point out, is that people like Branson often have huge (read: irrational) risk tolerances, and this fact becomes a factor in their success. One wonders whether Einstein, who lived a comfortable but certainly not rich life as a patent clerk, was entirely rational when he chose to take on the entire edifice of modern Newtonian physics with an untested theory.
Where would we be without these biases? And where would we be — if anywhere at all — if we adhered to Kahneman and Tversky’s injunction to be (axiomatically) rational? This takes us to AI.
How AI enthusiasts use natural stupidity to bolster claims of machine intelligence
Techno-futurists of all stripes — but in particular the true believers in a coming “singularity” or superintelligence most egregiously — attack human thinking as hopelessly biased, slow, and stupid. It’s no wonder they trumpet a coming machine intelligence with such ease — they’ve lowered the standards so much to begin with.
Kay and King here point out that the belief in machine intelligence assumes that radical uncertainty can be tamed: that it can be reduced or translated somehow into small worlds where the rules can be known and more data equals more visibility of likely outcomes. Echoing my own use of these terms, they describe this as a desire to convert mysteries into puzzles with known solutions:
Artificial Intelligence (AI) engages computers which can learn from experience. It is the means by which many believe that eventually all mysteries will become soluble puzzles.
Yet the AI crowd has things exactly backward. It’s not that AI will convert mysteries into puzzles that can then be solved, and thus — since machines are demonstrably better puzzle solvers than human brains — establish the superiority of machine intelligence over our own. No. It’s that, as Kay and King are at pains to point out, mysteries can’t be converted into puzzles, and this means that machine intelligence is at an extreme disadvantage exhibiting the sort of intelligence we value in the real world in which we live. This point is of extreme importance to understanding what is wrong with AI and thinking about the future of AI.
Mysteries are marked by the question, “What’s going on here?” The idea is that, since axiomatic rationality or in other words ratiocination won’t suffice to “solve” a mystery — we need insights, and abductive inferences rather than more data and faster processing — we need to develop a means to make plausible inferences and judgements in the absence of assignable probabilities. This is a game that computers don’t play particularly well (if at all). Since mysteries can’t be thought of as puzzles, and puzzle solving strategies are generally unfit for penetrating mysteries, we have a case of endemic artificial stupidity. Language models may have demonstrated that huge-scale puzzle solvers can convert the cyber world of words into intelligent output, but in the real world where we live words in cyberspace aren’t enough. That’s why self-driving cars are stuck at a stop light since roughly 2016. You can’t drive a ton of metal and plastic around in the real world using tricks in cyberspace.
Again, Kay and King (correctly) point out that small worlds versus large worlds (or axiomatic versus ecological rationality, or puzzles versus mysteries) inhabit what we might call different epistemic spaces. For humans with ecological rationality (was: cognitive biases), the different epistemic spaces of small and large world problems means that they’re in some sense “non-convertible” one to the other. The entire history of AI is one long and largely unsuccessful attempt to convert thought into a set of puzzles that can then be solved by various computational techniques.
No one builds an AI to “do abductive reasoning” because no one has the faintest clue how. It’s great that we built such large induction machines with LLMs that we can simulate some of the wonders of our human cognitive powers, if only by generating sequences of words in cyberspace. This is the very definition of puzzle-solving still, since one of the conditions of a puzzle (or a small world) is that more information reveals more of the solution. Or: more data and compute means better models. This is puzzle thinking.
The thought leaders in AI rarely admit that the larger plan here is something we already know is impossible: converting from small worlds to large worlds, or vice versa. The AI industry would rather trumpet successes on games, deep fakes, and text generation than confront the harsher reality of building something like Rosie the Robot or a fully autonomous vehicle. The news would be full of endless reports of failures and deeper and deeper problems.
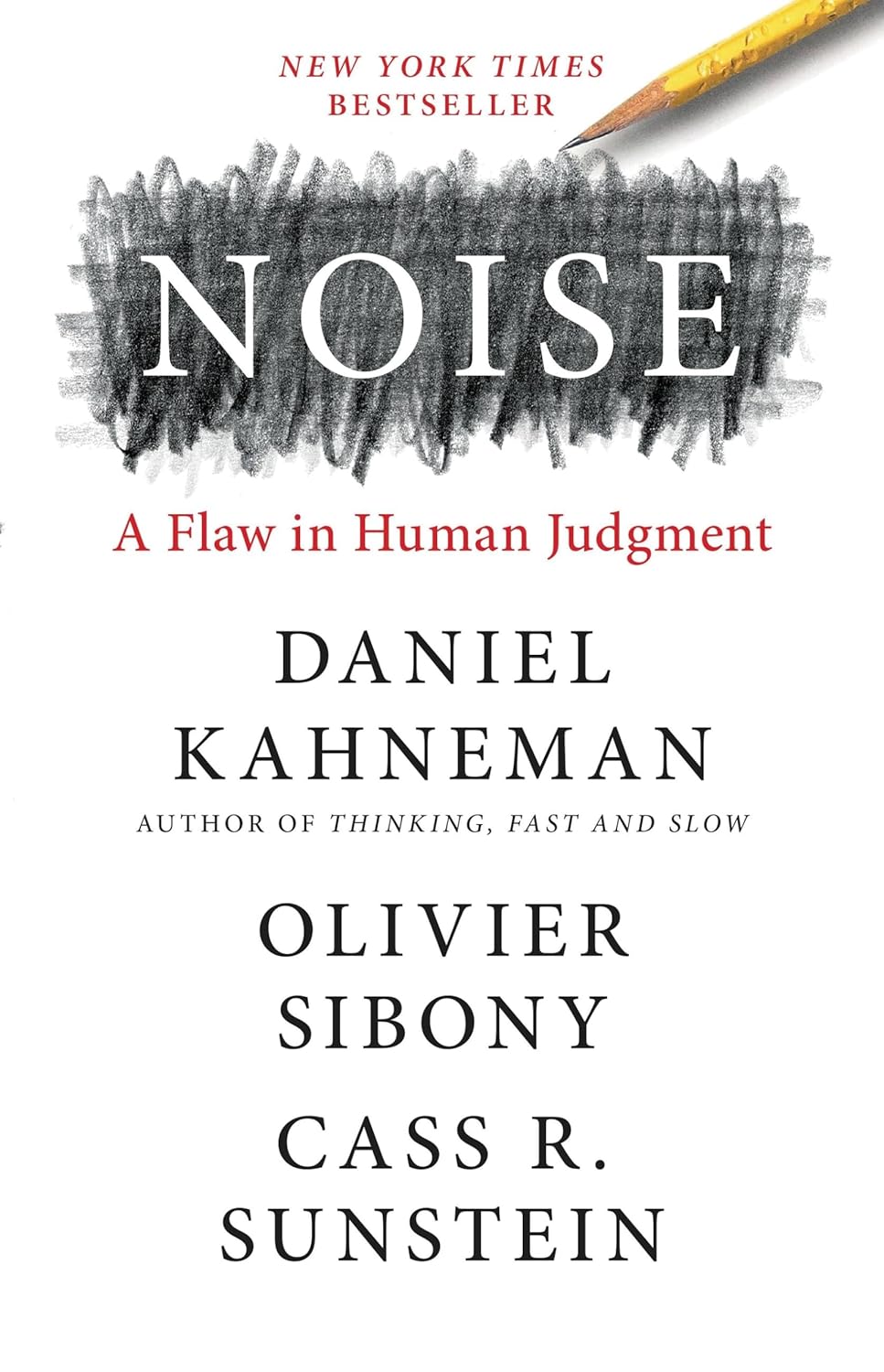
But it’s not silly for them to subtly downplay human potential with a full throated embrace of “natural stupidity” and cognitive bias. All this psychology and economics research perfectly props their goals.
Bad puzzle solvers?
Humans are clearly bad puzzle solvers compared to AI — look at chess, or now Go. We’re slow calculators, too, and we need to do things like eat and sleep. What we’re seeing here is an entire scientific paradigm — in the “soft sciences” — that buttresses the idea that humans are slow, crappy computers made of meat. How do we know? Look at the irrational responses to the Linda Problem! Look at all the biases!
Kay and King’s treatment of radical uncertainty in large (not small) worlds and our use of a more (not less) powerful form of thinking is important because it gives the lie to the techno-futurist’s arrogant and misinformed insistence that “it’s only a matter of time” before computers can do anything we can do, and better. Computers will keep calculating in the small worlds for which they are built. We’ll keep screwing up the Linda Problem and other silly tests as long as the research community is intent on denigrating human thinking rather than understanding it.
What’s “natural intelligence”? No one really knows. AI futurists will claim it either doesn’t exist, or it’ll be quickly in the rear view. As usual, they’re wrong. How we get out of this mess is an interesting mystery indeed.
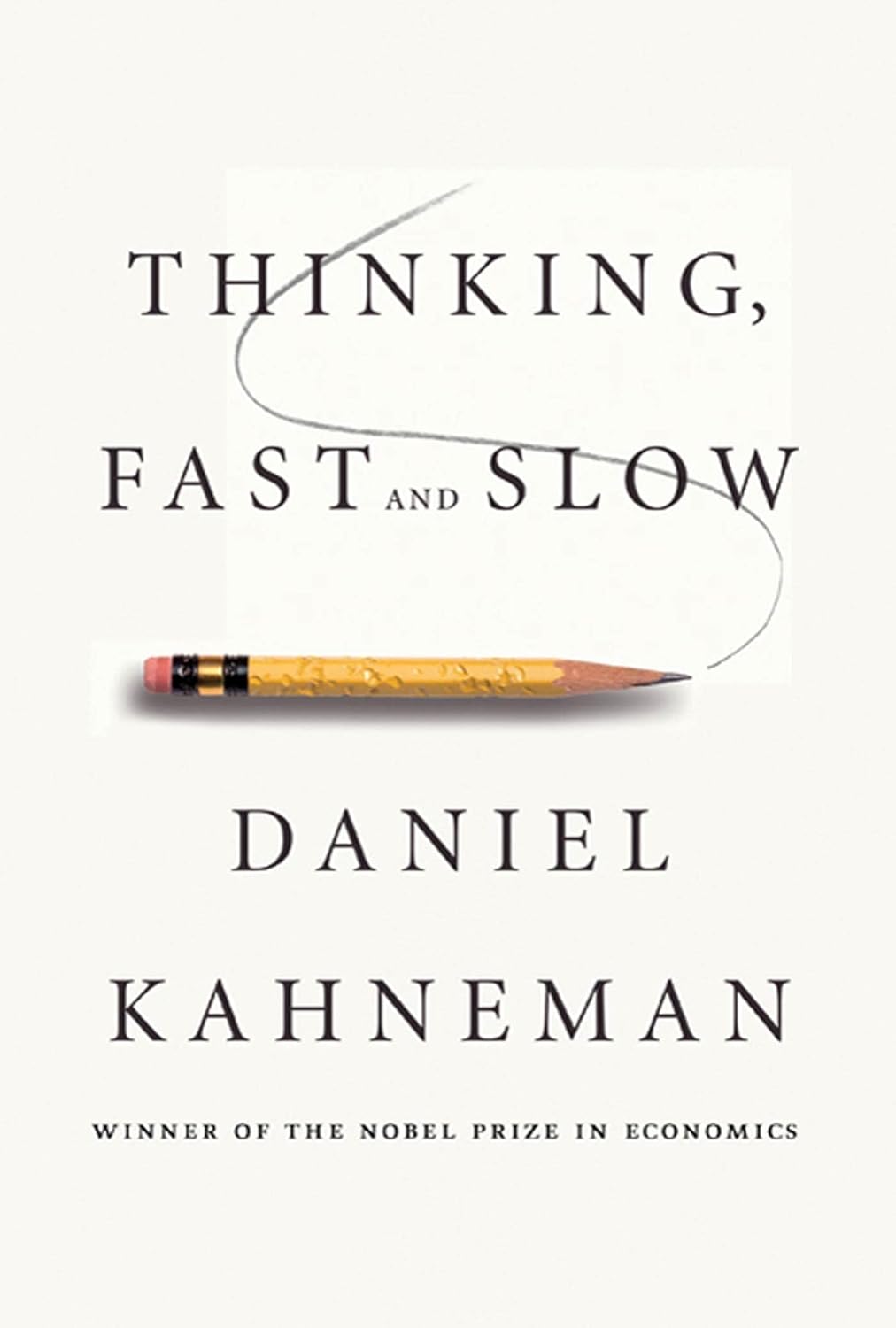
I’d like to close by mentioning Kahneman’s last book, published in 2021, Noise: A Flaw in Human Judgement, written with Olivier Sibony, a professor of strategy at HEC Paris. Its Amazon page highlights a quote: “Human judgment can therefore be described as measurement in which the instrument is a human mind.” Wrong-o. This must be a typo, because Human judgment precisely cannot be described as such. Not to pick on Kahneman, but his last salvo seems to have been another gross misunderstanding of ecological rationality.
The term “noise,” used in the title, is a statistical term that means random variability around a target. Judges who mete out different sentences for the same crimes depending on whether it’s before or after lunch are generating legal “noise.” But Kahneman again presupposes — really, sneaks in — small worlds where inconsistency can be calculated and a measure of noise versus signal makes sense. Many people read this latest book and concluded, as Kahneman did, that we should replace people making judgements with algorithms that generate less noise wherever possible. But humans will need to be out in front if we are to have any sort of future worth fighting for.
1 Kahneman describes the Linda Problem in his 2011 book Thinking, Fast and Slow. I’m following his description in that more recent work.