COVID-19, Bayes’ Rule, and Simpson’s paradox
Israeli data, when studied carefully, confirm the effectiveness of COVID-19 vaccinesIsrael has a very high COVID-19 vaccination rate and yet, on August 15, 2021, 58% of those Israelis hospitalized for COVID-19 were fully vaccinated — suggesting that vaccinations are ineffective or even harmful.
This is a great example of two common statistical traps. The first is confusion about inverse probabilities. One hundred doctors were once asked this hypothetical question:
In a routine examination, you find a lump in a female patient’s breast. In your experience, only 1 out of 100 such lumps turn out to be malignant, but, to be safe, you order a mammogram X-ray. If the lump is malignant, there is a 0.80 probability that the mammogram will identify it as malignant; if the lump is benign, there is a 0.90 probability that the mammogram will identify it as benign. In this particular case, the mammogram identifies the lump as malignant. In light of these mammogram results, what is your estimate of the probability that this lump is malignant?
Of the 100 doctors surveyed, 95 gave probabilities of around 75 percent — and you might be tempted to give a similar answer. However, the correct probability is only 7.5 percent, as shown by the following hypothetical data for 1,000 patients:
Test Positive | Test Negative | Total | |
Malignant | 8 | 2 | 10 |
Not Malignant | 99 | 891 | 990 |
Total | 107 | 893 | 1,000 |
The lump is malignant in 10 of the 1,000 cases (1 percent) and the mammogram gives a correct positive reading in 8 (80%) of these 10 cases. Of the 990 cases in which the lump is benign, the mammogram gives a correct negative reading for 891 (90%). There is a total of 107 positive readings, of which 99 are false positives. In only 8 (7.5%) of the 107 positive readings is the lump malignant.
The mathematics underlying these calculations is known as Bayes’ Rule, formulated by Thomas Bayes, an 18th century mathematician and minister. He was evidently trying to calculate the probability of God’s existence, given the way the world is, from the inverse probability that the world would be the way it is if there were a God. He didn’t have the information he needed to calculate that probability, but his efforts led him to what is now called Bayes’ Rule, which is invaluable for calculating inverse probabilities and for revising probabilities in the light of new information.
For the Israeli COVID-19 data, here is the relevant table:
Not Fully Vaccinated | Fully Vaccinated | Total | |
Hospitalized for COVID-19 | 214 | 301 | 515 |
Not Hospitalized for COVID-19 | 1,302,698 | 5,634,333 | 6,937,031 |
Total Population | 1,302,912 | 5,634,634 | 6,937,546 |
Probability Hospitalized | 0.00016425 | 0.00005342 | |
Risk Ratio | 3.07 |
Of the 515 persons hospitalized for COVID-19, the probability of being fully vaccinated is 301/515 = 0.584. Far more relevant is a comparison of the probability that a person who has been fully vaccinated is hospitalized with the same probability for people who are not fully vaccinated. As shown in the table, these probabilities are:
Probability hospitalized if not vaccinated = 214/1,302,912 = 0.00016425
Probability hospitalized if vaccinated = 301/5,634,634 = 0.00005342
The probability of being hospitalized is 3.07 times higher for those who are not vaccinated:
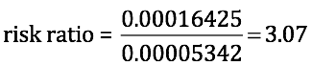
Even though those who were hospitalized were more likely to be vaccinated, those who were not vaccinated were far more likely to be hospitalized. The vaccine works!
Even these calculations are misleading because of the second statistical trap, Simpson’s Paradox, which occurs when a pattern in a set of data is reversed when the data are separated into subgroups. It was once reported that Alaska Airlines had a better on-time performance than another airline in every one of the five major airports they competed in, but a worse overall on-time record — because Alaska Air had many more flights into Seattle where weather problems frequently caused delays. Similarly, female mortality rates are lower in Sweden that in Costa Rica for every age group, but the overall female mortality rate is higher in Sweden — because Sweden has more elderly women (and the elderly have a relatively high death rate).
Here, we know that the elderly are more vulnerable to severe outcomes from COVID-19, so we should separate the data into age groups. A crude division is those under and over the age of 50:
Not Fully Vaccinated | Fully Vaccinated | Total | |
Under the Age of 50 | |||
Hospitalized for COVID-19 | 43 | 11 | 54 |
Not Hospitalized for COVID-19 | 1,116,791 | 3,501,107 | 4,617,898 |
Total Population | 1,116,834 | 3,501,118 | 4,617,962 |
Probability Hospitalized | 0.00003850 | 0.00000314 | |
Risk Ratio | 12.25 | ||
Over the Age of 50 | |||
Hospitalized for COVID-19 | 171 | 290 | 461 |
Not Hospitalized for COVID-19 | 185,907 | 2,133,226 | 2,319,133 |
Total Population | 186,078 | 2,133,516 | 2,319,594 |
Probability Hospitalized | 0.00091897 | 0.00013593 | |
Risk Ratio | 6.76 |
This is not a full-blown Simpson’s paradox in which probabilities are reversed when the data are disaggregated, but it has the same characteristics. The risk ratios for the unvaccinated are 12.25 for those under the age of 50 and 6.76 for those over the age of 50, but only 3.07 for the entire population because the unvaccinated are disproportionately younger and less vulnerable to COVID-19. The reality is that, young or old, the unvaccinated are much more likely to be hospitalized for COVID-19.
This table shows the risk ratios for a finer set of age ranges. (The risk ratios are infinite for those under the age of 30 because there were no vaccinated hospital patients under the age of 30.)
Age Range | Risk Ratio |
12-15 | ∞ |
16-19 | ∞ |
20-29 | ∞ |
30-39 | 31.25 |
40-49 | 16.39 |
50-59 | 13.89 |
60-69 | 8.85 |
70-79 | 9.62 |
80-89 | 5.29 |
90+ | 13.16 |
The proverbial bottom line is that the fact that nearly 60 percent of the people hospitalized for COVID-19 were fully vaccinated does not demonstrate that COVID-19 vaccines are ineffective. What these data do demonstrate is the importance of Bayes’ Rule and Simpson’s paradox. Instead of looking at the percent of hospitalized patients who were vaccinated, we should use Bayes’ Rule to determine the inverse probabilities — the chances that vaccinated and unvaccinated people will be hospitalized. In addition, when there is an important confounding factor like age, we need to consider Simpson’s Paradox. Here, for every age group, the risk ratios for the unvaccinated are far higher than the overall risk ratio. These Israeli data confirm the effectiveness of COVID-19 vaccines.